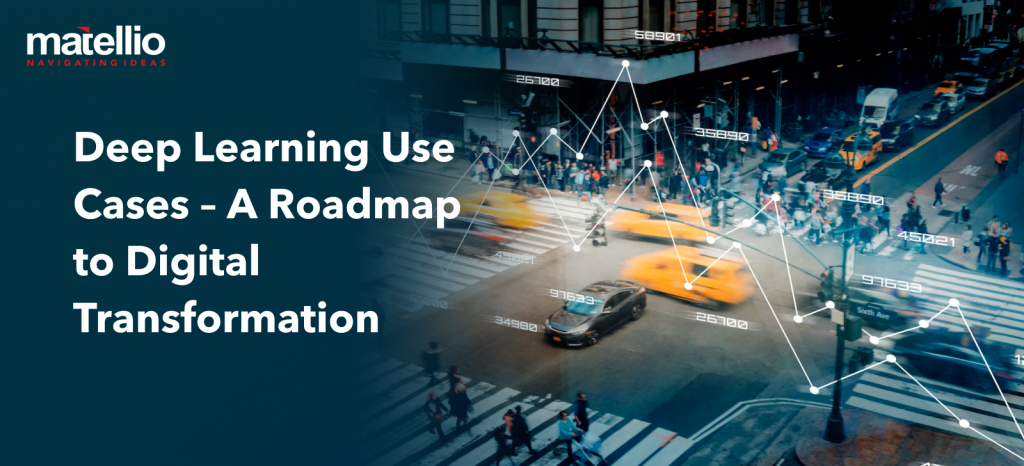
Have you ever wondered how your smartphone effortlessly recognizes your face or how your favorite streaming service recommends the perfect movie for your mood? Welcome to the era of Deep Learning, where machines not only understand but also learn from data, revolutionizing the way we interact with technology.
Deep Learning isn’t just a tech buzzword; it’s changing the game.
Whether it’s healthcare, finance, or even how your car drives itself, Deep Learning is making things better and smarter. It’s not about following fixed rules; it’s about machines learning patterns and getting better at what they do. Take natural language processing, for example; Deep Learning doesn’t just understand our words; it gets the context, feelings, and can even talk like us!
And guess what? We’re just getting started. Deep Learning is transforming industries and shaping the future, making our lives easier and more connected.
Continue reading as we explore the exciting Deep Learning business use cases that are reshaping how we live. Don’t miss out on the future – dive deep with us into the age of Deep Learning!
Why Invest in Deep Learning?
Deep learning, a subset of machine learning, is revolutionizing various industries with its ability to learn and make decisions based on complex data. Here’s a glimpse into its impact across different sectors:
The market for deep learning chips is expected to reach over 21 billion USD by 2027 due to its rapid growth.
With a predicted CAGR of 42.9% from 2022 to 2030, the deep learning software industry, which was valued at USD 5.46 billion in 2021, is expected to reach USD 133.56 billion.
Between 2022 and 2027, the US deep learning market is projected to develop at a compound annual growth rate (CAGR) of 29.19%.
Popular Deep Learning Use Cases
Popular deep learning use cases are found across diverse industries, highlighting how effective and adaptable deep learning algorithms are. Here are some key examples that illustrate their wide-ranging applications:
1. Image Recognition and Classification:
Deep Learning excels at recognizing and classifying images, powering applications like facial recognition, object detection, and visual search. This technology has found its way into security systems, social media platforms, and even medical imaging. Deep reinforcement learning use cases in image recognition and classification continue to evolve, shaping how we interact with visual information in various aspects of our lives.
2. Natural Language Processing (NLP):
NLP allows machines to understand, interpret, and generate human-like language. Deep Learning models in this domain are behind virtual assistants, chatbots, language translation services, and sentiment analysis tools. These deep learning use cases in NLP significantly impact our communication with technology, making it more natural and accessible.
3. Fraud Detection in Finance:
Financial institutions leverage Deep Learning to detect and prevent fraudulent activities. By analyzing transaction patterns, user behavior, and identifying anomalies, these models enhance security and protect against financial fraud. Deep learning use cases in finance play a crucial role in safeguarding financial transactions and maintaining trust in the digital economy.
5. Recommendation Systems:
Deep Learning powers recommendation engines in platforms like Netflix, Amazon, and Spotify. By analyzing user behavior and preferences, these systems suggest personalized content, products, or music, enhancing user experience and engagement. Deep learning use cases in recommendation systems contribute to the customization of online experiences, making content discovery more tailored to individual preferences.
6. Generative Adversarial Networks (GANs) in Creativity:
GANs, a type of Deep Learning model, are used for creative tasks such as image generation, style transfer, and even the creation of realistic artwork. These models contribute to the intersection of technology and art. Deep learning use cases in creativity showcase the potential for AI to augment and inspire human creativity across various artistic domains.
7. Financial Market Forecasting:
Deep learning models, powered by AI Integration Services, analyze historical market data, news sentiment, and global events to make predictions about financial markets. Traders and investors use these insights to make informed decisions in the dynamic world of finance. These use cases in financial market forecasting underscore AI’s role in providing valuable insights for navigating the complexities of the financial landscape.
8. Customer Service Chatbots:
Deep Learning powers intelligent chatbots that enhance customer service by providing instant responses, answering queries, and resolving issues. These chatbots are increasingly becoming an integral part of online customer support. Deep learning use cases in customer service showcase how AI-driven interactions contribute to improved customer experiences and streamlined support services.
These use cases demonstrate the versatility of Deep Learning, showcasing its impact across daily life activities. As technology continues to advance, we can expect even more innovative applications of Deep Learning in the future.
For businesses seeking to stay ahead in this dynamic landscape, implementing Deep Learning as part of their enterprise solutions with Matellio becomes not just a choice but a strategic imperative.
Industry-based Deep Learning Use Cases: Impact and Adoption
Deep Learning, with its ability to extract intricate patterns from vast datasets, has become a transformative force across various industries. Let’s delve into specific applications within key sectors, where Deep Learning is reshaping operations, enhancing efficiency, and fostering innovation.
Deep Learning Use Cases in Banking:
Fraud Detection:
Deep Learning algorithms scrutinize transaction patterns, flagging suspicious activities and potential fraud in real-time. They analyze vast amounts of data, including transaction history, user behavior, and geographic location, to identify anomalies and fraudulent patterns with high accuracy. Deep learning use cases in banking play a crucial role in safeguarding financial transactions and maintaining trust in the banking ecosystem.
Customer Service Chatbots:
Intelligent chatbots powered by Deep Learning provide personalized assistance, improving customer interactions and query resolutions. These chatbots utilize natural language processing (NLP) algorithms to understand user inquiries and offer relevant solutions promptly, enhancing customer satisfaction and reducing service costs.
Credit Scoring:
Predictive models analyze diverse data sources to assess creditworthiness, streamlining the credit approval process. Deep Learning algorithms leverage customer demographics, financial history, and behavioral patterns to generate accurate credit scores, enabling financial institutions to make informed lending decisions efficiently.
Risk Assessment:
Deep learning models evaluate financial risks by analyzing market trends and historical data, ensuring robust risk assessment and mitigation strategies. They leverage complex algorithms to identify potential risks, such as market fluctuations or credit defaults, enabling proactive risk management and regulatory compliance.
Deep Learning Use Cases in Healthcare:
Medical Imaging:
Deep Learning excels in the analysis of medical images, aiding in the early detection of diseases through precise interpretation of X-rays, MRIs, and CT scans. These algorithms detect subtle abnormalities and lesions, assisting healthcare professionals in accurate diagnosis and treatment planning, ultimately improving patient outcomes. Deep learning use cases in healthcare extend to medical imaging, where AI-driven analysis enhances diagnostic accuracy and efficiency.
Drug Discovery:
Accelerating drug development by predicting molecular interactions and identifying potential candidates for new medications. Deep Learning algorithms analyze vast molecular datasets, predicting drug-target interactions and identifying promising compounds, expediting the drug discovery process and reducing research costs.
Personalized Medicine:
Deep Learning models utilize patient data to tailor treatment plans, ensuring more targeted and effective healthcare interventions. By analyzing genetic, genomic, and clinical data, these models identify personalized treatment options, optimizing therapeutic outcomes and minimizing adverse effects.
Disease Prediction:
Deep learning algorithms analyze patient data to predict the likelihood of developing certain diseases, enabling proactive healthcare management. By integrating clinical data, genetic markers, and lifestyle factors, these models assess disease risk, facilitating early intervention and preventive care strategies.
Also Read: Discover how machine learning in drug discovery can accelerate pharmaceutical innovation with custom AI solutions, optimizing research and enhancing drug development efficiency.
Deep Learning Use Cases in Finance:
Algorithmic Trading:
Deep Learning models analyze market trends and historical data to develop algorithmic trading strategies, optimizing investment decisions. They leverage advanced machine learning algorithms to identify profitable trading opportunities, enhance portfolio performance, and mitigate market risks.
Risk Management:
Identifying and mitigating financial risks through advanced analytics, ensuring robust risk assessment and mitigation strategies. Deep learning algorithms analyze complex financial data, including market volatility, credit risk, and liquidity metrics, to identify potential risks and vulnerabilities, enabling proactive risk management and regulatory compliance.
Customer Relationship Management:
Leveraging customer data to provide personalized financial advice, enhancing the overall customer experience. Deep learning algorithms analyze customer preferences, behaviors, and transaction history to offer tailored financial products and services, fostering customer loyalty and retention.
Fraud Prevention:
Deep learning algorithms analyze transaction patterns and customer behavior to detect and prevent fraudulent activities, safeguarding financial institutions and customers alike. By leveraging advanced analytics and anomaly detection techniques, these models identify suspicious transactions and activities in real-time, mitigating financial losses and reputational risks.
Read More: Revolutionize Your Business with Deep Learning in Financial Services
Deep Learning Use Cases in Automotive:
Autonomous Driving:
Deep Learning enables vehicles to navigate and make decisions autonomously, revolutionizing the automotive industry. These algorithms analyze real-time sensor data, including LiDAR, radar, and cameras, to perceive the surrounding environment and navigate safely.
Predictive Maintenance:
Anticipating and preventing mechanical failures by analyzing real-time data from sensors, reducing downtime and maintenance costs. Deep Learning algorithms analyze sensor data from vehicles to detect anomalies and predict potential equipment failures, enabling proactive maintenance interventions.
Image and Object Recognition:
Enhancing safety through the detection of pedestrians, obstacles, and road signs using advanced computer vision. Deep Learning models process visual data captured by cameras to detect and classify objects in the vehicle’s environment, improving driver awareness and accident prevention.
Natural Language Processing:
Deep learning techniques are employed for speech recognition and natural language understanding in automotive interfaces, enhancing user experience and safety by enabling hands-free communication and intelligent voice commands.
Deep Learning Use Cases in Insurance:
Claims Processing:
Streamlining and automating the assessment and validation of insurance claims through image and document analysis. Deep Learning algorithms extract information from documents and images, automating claim processing workflows and reducing manual intervention.
Risk Assessment:
Deep Learning models predict and evaluate risks associated with insurance policies, aiding in precise underwriting. These models analyze diverse data sources, including customer demographics, property information, and historical claims data, to assess risk factors and determine appropriate premiums.
Fraud Detection:
Identifying fraudulent activities by analyzing patterns and anomalies in insurance data, enhancing overall security. Deep learning algorithms leverage advanced analytics and anomaly detection techniques to identify suspicious claims and behaviors, enabling timely intervention and fraud prevention.
Customer Segmentation:
Deep learning algorithms segment customers based on their insurance needs and behaviors, allowing for targeted marketing and personalized services. By analyzing customer demographics, preferences, and past interactions, insurers can tailor their products and services to meet the specific needs of different customer segments.
Read More: Deep Learning in Insurance: The Key to a More Efficient and Effective Business
Deep Learning Retail Use Cases:
Inventory Management:
Optimizing stock levels and reducing wastage through predictive analytics and demand forecasting. Deep Learning algorithms analyze historical sales data, market trends, and external factors to forecast demand accurately, enabling retailers to optimize inventory levels and reduce carrying costs.
Read More: Unlock the potential of retail planogram software to streamline product placement, drive customer engagement, and boost sales using deep learning innovations.
Customer Segmentation:
Tailoring marketing strategies by analyzing customer behavior and preferences, improving targeting and engagement. Deep Learning models analyze customer transaction data, browsing history, and social media interactions to segment customers into distinct groups based on their preferences and purchasing habits.
Visual Search:
Enhancing the customer experience by allowing visual search capabilities, making product discovery more intuitive. Deep Learning algorithms analyze product images and user queries to enable visual search functionality, allowing customers to search for products using images rather than text.
Recommendation Systems:
Deep learning algorithms analyze customer purchase history and preferences to offer personalized product recommendations, increasing sales and customer satisfaction. By leveraging collaborative filtering and content-based recommendation techniques, retailers can suggest relevant products to customers based on their preferences and browsing behavior.
Deep Learning Use Cases in Manufacturing:
Quality Control:
Automated inspection using computer vision ensures product quality by identifying defects and inconsistencies. Deep Learning algorithms analyze images captured by cameras to detect defects and anomalies in manufactured products, ensuring compliance with quality standards.
Predictive Maintenance:
Anticipating equipment failures by analyzing sensor data, minimizing downtime and improving overall operational efficiency. Deep Learning models analyze sensor data from machinery and equipment to detect patterns indicative of potential failures, enabling proactive maintenance and minimizing unplanned downtime.
Supply Chain Optimization:
Deep Learning optimizes the supply chain by predicting demand, reducing costs, and improving overall efficiency. By analyzing historical sales data, market trends, and external factors, these models optimize inventory levels, production schedules, and distribution routes to meet customer demand while minimizing costs.
Process Optimization:
Deep learning models optimize manufacturing processes by analyzing data from sensors and production lines, improving efficiency and reducing waste. By monitoring key process parameters and identifying optimization opportunities, these models enable manufacturers to streamline operations and improve productivity.
Read More: Deep Learning in Manufacturing: Transforming Your Business
Deep Learning Use Cases in Telecom:
Network Security:
Identifying and preventing cybersecurity threats through advanced threat detection and analysis. Deep Learning algorithms analyze network traffic patterns and behavior to detect and mitigate cyber threats, protecting against malware, intrusions, and data breaches.
Predictive Analytics for Network Maintenance:
Anticipating and addressing network issues proactively to ensure uninterrupted connectivity. Deep Learning models analyze historical network performance data and predict potential failures, enabling telecom operators to schedule maintenance and upgrades proactively.
Read More: Predictive Analytics in Telecom
Customer Churn Prediction:
Analyzing customer data to predict and prevent churn, enabling targeted retention strategies. Deep Learning algorithms analyze customer behavior, usage patterns, and service interactions to identify customers at risk of churn and recommend targeted retention offers and interventions.
Network Optimization:
Deep learning algorithms optimize network traffic and resource allocation to improve performance and user experience. By analyzing network data and user behavior, these models optimize bandwidth allocation, routing, and QoS parameters to enhance network performance and reliability.
Read More: Telecom Analytics
These use cases of deep learning demonstrate the adaptability and transformative potential of this technology across diverse industries, shaping the future of how businesses operate and innovate. For organizations seeking to embrace the future, Digital transformation services can play a pivotal role in integrating and optimizing Deep Learning solutions within their existing frameworks.
Also Read: Discover how AI in Disease Diagnosis is revolutionizing healthcare by merging cutting-edge innovation with business excellence to deliver faster, more accurate, and cost-effective diagnostic solutions.
Why Choose Deep Learning for Your Business: Challenges with Solutions
Deep learning, with its sophisticated neural network architectures and advanced learning algorithms, offers profound solutions to various challenges across different facets of data, model, and optimization in machine learning tasks.
Challenge: Insufficient Data
Deep learning excels in learning representations from large volumes of data. It can alleviate the impact of insufficient data through techniques such as data augmentation, where existing data is manipulated to create new samples, and transfer learning, which leverages knowledge from pre-trained models on similar tasks. Additionally, deep learning can generate synthetic data to supplement training sets and employ active learning strategies to selectively label the most informative samples, optimizing data collection efforts.
Challenge: Imbalanced Classes
Deep learning models can address class imbalances by employing various techniques. Resampling methods, such as oversampling minority classes or undersampling majority classes, rebalance the dataset. Algorithms like Synthetic Minority Over-sampling Technique (SMOTE) generate synthetic samples to balance class distributions. Cost-sensitive learning assigns different misclassification costs to different classes, effectively managing imbalances.
Challenge: Noisy Data
Deep learning models are robust to noise in data due to their ability to learn complex patterns. Preprocessing techniques like noise removal and normalization can help clean up noisy data. Outlier detection and removal methods ensure that the model focuses on relevant patterns. Additionally, robust model architectures with regularization techniques can mitigate the impact of noisy inputs.
Challenge: Overfitting and Underfitting
Deep learning offers solutions to combat overfitting and underfitting. Regularization techniques such as L1 and L2 regularization and dropout prevent over-reliance on specific features and encourage generalization. Cross-validation helps assess model performance and prevent overfitting by evaluating models on multiple subsets of the data. Early stopping prevents overfitting by terminating training when performance on a validation set starts to degrade.
Challenge: Vanishing or Exploding Gradients
Deep learning models tackle the problem of vanishing or exploding gradients using different methods. Gradient clipping controls the size of gradients during training to avoid explosions. Using suitable activation functions such as ReLU helps reduce the vanishing gradient issue. Normalizing inputs and using improved weight initialization techniques ensure a steady gradient flow during training.
Challenge: Model Interpretability
Deep learning models offer ways to understand how they make decisions using interpretability techniques. Methods like feature importance show how much each feature contributes to predictions. Tools such as LIME (Local Interpretable Model-agnostic Explanations) explain individual predictions. Saliency maps help visualize the important parts of input data for better understanding the model’s output.
Challenge: Optimization
Deep learning deals with slow training by speeding up computations using hardware acceleration, such as Graphics Processing Units (GPUs) or Tensor Processing Units (TPUs), and distributed training across multiple devices or machines. Improving code through vectorization and parallelization helps accelerate computations. Choosing the right batch sizes balances computational efficiency with model performance. Profiling and optimizing operations ensure resources are used efficiently.
Challenge: Hyperparameter Tuning
Deep learning uses different methods to adjust hyperparameters. Grid search looks at every possible combination in a set range. Random search picks hyperparameters randomly within a given range. Bayesian optimization understands how changes affect performance and picks the best hyperparameters. Automated machine learning (AutoML) tools handle hyperparameter tuning automatically, finding the best settings faster.
By harnessing the power of deep learning, you can derive valuable intelligence from your data, make informed decisions, and gain a competitive edge in your respective industry. Additionally, the expertise and guidance of our specialized technology consulting services can further enhance the implementation of deep learning solutions, ensuring alignment with organizational goals and maximizing the impact of machine learning initiatives.
Also Read: Explore how LLM model comparison can help you choose the best AI solution for your business, enhancing efficiency and driving smarter decision-making.
Implement Deep Learning Use Cases with Matellio
In a world where innovation is the heartbeat of success, integrating Deep Learning Use Cases is not just a choice but a strategic imperative for businesses. From enhancing customer experiences to optimizing operations, Deep Learning opens new dimensions of efficiency and foresight.
As your venture navigates this era of technological evolution, partnering with Matellio, a leading AI Development Company, ensures seamless integration of cutting-edge solutions tailored to your unique needs.
Our expertise in developing and implementing Deep Learning Use Cases empowers your business with predictive analytics, personalized customer interactions, and operational excellence.
Whether you’re in finance, healthcare, manufacturing, or any other sector, our AI-driven solutions are designed to elevate your operations, drive innovation, and stay ahead in a competitive landscape.
From fraud detection in banking to predictive maintenance in manufacturing, we craft solutions that align seamlessly with your objectives. As your trusted partner, we don’t just implement technology; we pave the way for your business to thrive in the digital age.
Let’s innovate together, because at Matellio, we don’t just develop solutions; we engineer success for your business in the age of AI.
Deep Learning Use Cases – FAQs
Q1: What is Deep Learning, and how does it differ from traditional machine learning?
Deep Learning is a subset of machine learning that involves neural networks with multiple layers (deep neural networks). Unlike traditional machine learning, deep learning automatically learns hierarchical representations of data, allowing for more complex and intricate pattern recognition.
Q2: How can Deep Learning benefit my business?
Deep Learning offers various benefits, including enhanced data analysis, improved decision-making processes, and the ability to automate complex tasks. It can optimize operations, personalize customer experiences, and provide valuable insights for strategic planning.
Q3: What industries can benefit from implementing Deep Learning Use Cases?
Deep Learning has versatile applications across industries such as healthcare, finance, automotive, retail, manufacturing, and more. Its adaptability makes it a valuable tool for optimizing processes, improving efficiency, and fostering innovation.
Q4: What is the role of Matellio as an AI Development Company in implementing Deep Learning solutions?
Matellio specializes in developing and implementing Deep Learning solutions tailored to the unique needs of businesses. As an AI Development Company, Matellio ensures seamless integration of cutting-edge technology, empowering businesses with predictive analytics, personalized interactions, and operational excellence.
Q5: Is Deep Learning only applicable to large enterprises, or can small businesses benefit as well?
Deep Learning is scalable and adaptable, making it suitable for businesses of all sizes. Small businesses can leverage its capabilities to enhance efficiency, automate tasks, and gain a competitive edge.
Q6: What are the key considerations when implementing Deep Learning Use Cases in a business?
Key considerations include defining clear objectives, ensuring data quality, having the right infrastructure, and selecting the appropriate algorithms. Collaborating with experienced AI development partners like Matellio can also streamline the implementation process.