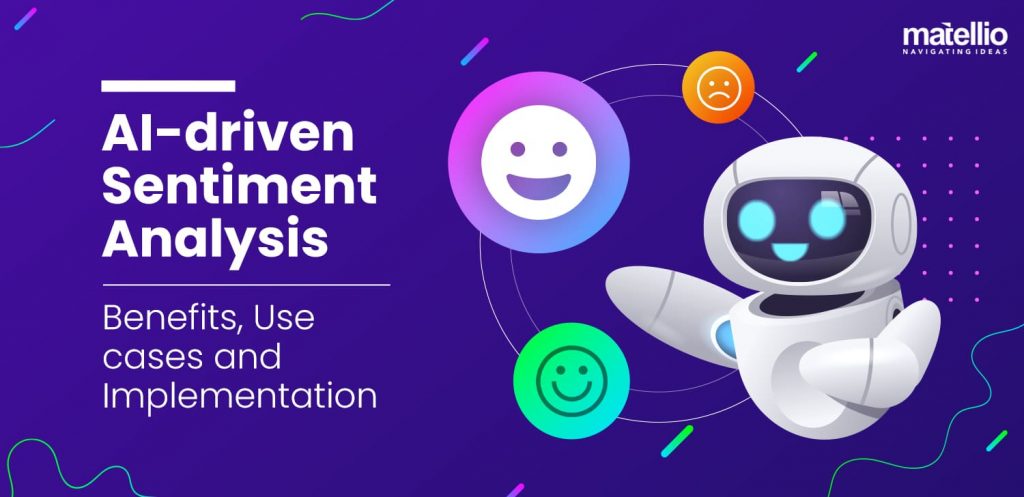
A household name since the 2019 pandemic, Zoom is now garnering headlines again, this time for its new project around sentiment analysis. The company is investing in an emotion-analyzing system that will evaluate the engagement depth and emotions of the users during their online calls. This is not the first time a corporate is investing in this futuristic AI application. Companies like Apple, KFC, Twitter, and TripAdvisor have been using sentiment analysis applications to improve their customer experience and then eventually their services and products for increased revenues.
Thanks to the long-term investments by such conglomerates, AI-driven sentiment analysis has already become a market worth USD 3.15 billion in 2021 and is forecasted to grow at a CAGR of 14.4% by 2030. In this post, we will uncover how different industries are using the technology, what benefits they expect from its application, and how a business can implement the same to future-proof their customer experience.
What is Sentiment Analysis?
So, before diving into the ocean of sentiment analysis applications, let us first understand what it actually is. Sentiment analysis is an application of AI that uses natural language processing (NLP) services and machine learning algorithms to discern and understand the sentiment or emotional tone conveyed within textual data. This AI technology extracts meaningful information, from unstructured text, such as customer reviews, social media posts, surveys, and more, to detect sentiment, attitudes, emotions, and opinions. Intrigued?
Sentiment analysis applications also aim to understand the nuanced nuances of human language, including sarcasm, irony, and context-dependent sentiments. Feeling overwhelmed with this technical information? Let’s learn what sentiment analysis is from a simple example!
Imagine a big retail chain that operates both brick-and-mortar stores and an extensive online marketplace. Now, the company regularly collects feedback and reviews from its customers via different channels, such as feedback forms, online reviews, social media posts, and even customer service interactions.
But do you think they have the time and resources to manually sift through thousands of customer reviews and social media comments to gauge sentiment and identify actionable insights? This is where sentiment analysis comes into play!
Using sentiment analysis tools and techniques, the retail corporation can automatically analyze and categorize customer feedback into positive, negative, or neutral sentiments.
This way, the retail corporation can gain valuable insights into customer perceptions, identify emerging trends or issues, and take proactive measures to address customer concerns.
For example, a sentiment analysis application would help the retail store to identify recurring complaints about a specific product feature or delivery delay and take corrective actions, such as product improvements or process optimizations, to enhance customer experiences and drive loyalty.
Similarly, sentiment analysis applications can also be used in corporates to analyze employee sentiments and detect any signs of dissatisfaction or morale issues and intervene proactively to prevent escalation.
Read More: How can AI-driven Sentiment Analysis Help Improve Your Brand?
How Does Sentiment Analysis Work?
So, now that you understand the term sentiment analysis, you will probably be thinking about how sentiment analysis applications work. What process do they follow, what technology do they use, and how do they detect the actual sentiments from unstructured data? Let’s explore!
Sentiment analysis might seem like magic, but it’s powered by a fascinating combination of technology and artificial intelligence. The process of sentiment analysis involves several steps, including text preprocessing, feature extraction, sentiment classification, and analysis. Machine learning models, such as Naive Bayes, Support Vector Machines (SVM), and Recurrent Neural Networks (RNN), are commonly employed to automate this process and classify text sentiment accurately. Let’s dive into the process for a better understanding:
Data Collection
The journey begins with web crawlers and API integrations, which scour social media platforms, review sites, and other online sources to gather relevant text data like customer reviews, comments, and survey responses.
Text Preprocessing
Raw data is messy! Here, Natural Language Processing (NLP) techniques come into play. NLP tools clean and prepare the text for analysis. Imagine this as a linguistic spring cleaning – removing punctuation, correcting typos, and even recognizing slang or informal language.
Sentiment Scoring
Now comes the heart of the operation: Machine Learning (ML) algorithms. These algorithms, trained on massive datasets of text labeled with sentiment (positive, negative, or neutral), analyze the cleaned text. They consider factors like word choice, sentence structure, and even emojis to assign a sentiment score to each piece of data.
Analysis and Insights
Finally, the magic happens! The sentiment scores from all the analyzed text are aggregated and visualized using data analysis tools. This unveils the bigger picture – overall customer sentiment towards your brand, specific products, or marketing campaigns.
This comprehensive analysis empowers you to make data-driven decisions and truly understand the voice of your customer.
Types of Sentiment Analysis that You Can Leverage for Your Business
Well, knowing sentiment analysis isn’t enough to plan the implementation process.
You have to choose the best type of sentiment analysis for your business depending on your specific objectives, the nature of your textual data, and the level of granularity required for sentiment analysis. It’s essential to evaluate the strengths and limitations of each type of sentiment analysis and select the one that aligns most closely with your business needs and objectives.
Let’s delve into each type of sentiment analysis:
1. Document-level Sentiment Analysis
Document-level sentiment analysis, also known as document-level polarity detection, involves analyzing the sentiment expressed across an entire document, such as a review, article, or social media post. This type of sentiment analysis provides an overall assessment of the sentiment conveyed in the text, whether it’s positive, negative, or neutral.
It is useful for understanding the overall sentiment trends and opinions expressed in large volumes of text data, such as customer reviews, product feedback, or social media discussions.
2. Sentence-level Sentiment Analysis
Sentence-level sentiment analysis focuses on analyzing the sentiment expressed within individual sentences or phrases within a document. It enables a more granular understanding of sentiment nuances at the sentence level, allowing businesses to identify specific sentiments expressed within complex or lengthy texts.
This type of sentiment analysis is beneficial for dissecting text data into smaller units and extracting sentiment insights at a more detailed level.
3. Aspect-based Sentiment Analysis
Aspect-based sentiment analysis, also known as aspect-based opinion mining, goes beyond document or sentence-level analysis to identify sentiments related to specific aspects or features within a document. It aims to determine the sentiment polarity associated with various aspects or attributes of a product, service, or topic mentioned in the text.
This type of sentiment analysis application is particularly valuable for businesses seeking to understand customer opinions about specific features or attributes of their products or services and pinpoint areas for improvement.
4. Entity-level Sentiment Analysis
Entity-level sentiment analysis focuses on extracting sentiment polarity associated with named entities mentioned in the text, such as products, brands, organizations, or individuals.
The sentiment analysis applications built on this type can easily identify the sentiment expressed towards specific entities within the text and categorize it as positive, negative, or neutral. This type of sentiment analysis is useful for businesses to monitor and analyze customer perceptions and sentiments towards their brand, products, or competitors mentioned in textual data.
5. Fine-grained Sentiment Analysis
Fine-grained sentiment analysis involves assigning sentiment scores to text based on a finer granularity of sentiment categories beyond just positive, negative, or neutral. It may involve categorizing sentiments into multiple levels, such as very positive, positive, neutral, negative, or very negative, allowing for a more nuanced understanding of sentiment intensity.
This type of sentiment analysis application provides businesses with richer insights into the varying degrees of sentiment expressed in textual data, enabling more precise sentiment analysis.
6. Multilingual Sentiment Analysis
Multilingual sentiment analysis is capable of analyzing sentiment in text data written in multiple languages. It utilizes techniques to handle text data in different languages and accurately determine sentiment polarity across diverse linguistic contexts.
This type of sentiment analysis is beneficial for businesses operating in global markets or dealing with multilingual customer feedback, allowing them to gain insights into sentiment trends across different language demographics.
7. Emotion Detection
Last but not least, we have emotion detection, also known as sentiment analysis with emotion recognition that focuses on identifying and categorizing the emotions expressed in text data. It goes beyond sentiment polarity to detect specific emotions such as joy, sadness, anger, fear, or surprise conveyed in textual content.
This type of sentiment analysis enables businesses to understand the emotional context of customer feedback, social media posts, or online reviews, providing deeper insights into consumer sentiment and behavior.
Why Invest in Sentiment Analysis Application Development?
In today’s fiercely competitive business landscape, understanding your customers is no longer enough. You need to hear their voice, understand their emotions, and anticipate their needs.
That’s the reason why the market for sentiment analysis is expected to reach $6.8 billion by 2031! And that’s not all. Here are some more market stats that facilitate the adoption of sentiment analysis applications by companies globally.
From the stats mentioned above, one thing is clear that investing in sentiment analysis application development can provide numerous benefits and competitive advantages for your company. Here’s why your company should consider making this investment:
Transform Raw Data into Actionable Insights
Sentiment analysis applications unlock the hidden potential within mountains of customer feedback data. They translate emotions behind words, allowing you to identify trends, address concerns, and capitalize on opportunities.
Enhanced Customer Understanding
By leveraging the applications of sentiment analysis, you can analyze customer feedback from various sources such as social media, reviews, surveys, and customer support interactions. All that helps you to better understand what your customers like, dislike, and feel about your products or services.
Boost Customer Satisfaction and Loyalty
Sentiment analysis empowers you to identify and address customer pain points before they escalate into major issues. This proactive approach fosters stronger customer relationships and builds brand loyalty.
Gain a Competitive Advantage
Sentiment analysis empowers you to stay ahead of competitors by monitoring and analyzing customer sentiment towards your products, services, and competitors. By identifying emerging trends, market insights, or competitive threats, you can make informed business decisions, refine your strategies, and differentiate your offerings in the market.
Product and Service Optimization
Sentiment analysis provides valuable feedback on product features, quality, performance, and user experience. By analyzing sentiment data, you can identify areas for product or service improvement, prioritize feature enhancements, and tailor your offerings to better meet customer needs and preferences.
Marketing and Brand Management
With a custom AI sentiment analysis application, you can easily measure the effectiveness of your marketing campaigns, brand messaging, and communication strategies. By monitoring sentiment trends, sentiment towards your brand, and reactions to marketing initiatives, you can refine your marketing efforts, optimize messaging, and strengthen brand perception.
Risk Mitigation for Your Company
Sentiment analysis enables you to detect and mitigate potential reputation risks, crises, or negative publicity in real-time. By monitoring sentiment across social media, news articles, and online forums, you can identify early warning signs of negative sentiment or brand sentiment shifts and take proactive measures to address issues before they escalate.
In short, investing in sentiment analysis application development is not just about keeping a pulse on customer sentiment; it’s about unlocking a treasure trove of insights that can fuel your business growth and success. The market is recognizing the value of sentiment analysis as well. So, why should you lag? Contact us for a free 30-minute consultation to implement sentiment analysis in your business!
Top 15 Applications of Sentiment Analysis for Businesses in 2024 and Beyond
So, we are at the most awaited section of our blog post – exploring the robust applications of sentiment analysis for businesses. Today, the power of sentiment analysis extends far beyond simply gauging positive or negative feedback. Businesses globally are leveraging sentiment AI for custom enterprise software development to gain a deeper understanding of their customers across various touchpoints.
Here are 15 transformative use cases of sentiment analysis that can empower your business for success:
Retail
Retail businesses can use technology to identify trends and gauge how consumers feel about their brand. Deloitte has been working on the use cases for the largest retail franchises in South Africa.
The company analyzed customers’ sentiments over social media channels, figuring out that most negative emotions around the retailers are about the turnaround time of their support services. The analysis also helped the chains understand the upcoming trends like innovative technologies like NFC and solutions supporting sustainability. Both are huge investments, and the retailers hesitated to take the step until the sentiment analysis algorithm clarified all their doubts.
Similarly, a clothing retail giant has been using the technology to derive in-depth semantic insights from social media websites and user-generated videos to recognize trends early on. That has given the company a competitive edge, and a more comprehensive look into customer behavior segregated based on geography, language, age, gender, etc.
Tourism and Hospitality
In travel and hospitality, the tool is mostly used to evaluate guest reviews to understand what aspects of the business are most valuable to the guests and which cause them the most issues. This way, they can leverage their stronger aspects to market their products and services to a similar audience set. On the other hand, they can also prioritize fixing the problems that most customers face and express negative feelings about.
Other than that, the travel and hospitality businesses can also ascertain what services/amenities are doing well with the guests and which are not. That way, they can invest more resources in the ones eliciting positive responses from guests and skip the ones that bring little difference to their experience but cost the business money.
The companies already benefiting from adopting the above-mentioned use cases are Travel Media Group, Sentiment140, etc. Now considering how the pandemic-induced conditions have affected the industry in recent years, adopting such avant-garde solutions can help them gain and retain customer loyalty, the real currency in travel & hospitality.
Healthcare
Sentiment analysis is quickly becoming increasingly popular in the healthcare industry thanks to its ability to measure the effectiveness of different accreditations, medical staff training, and the effects of new measures on patients’ comfort.
Most applications in healthcare use sentiment analysis to detect problems and strengths just like a business in any sector would; what’s different here is the complexity involved.
Words used in clinical narratives are quite different than in the other sector. Even the social media clinical conversations are different than the actual conversations on the field, the latter consisting mostly of nouns and body locations. That can lead to a less subjective use of language in the field, making sentiment analysis more niche and complex.
Telecommunications
If there’s any sector that can benefit the most from sentiment analysis technologies, it has to be telecommunications. After all, the entire industry’s success depends on how well they handle consumers’ demands, complaints, and experience requirements. One popular example from the industry comes from a big European mobile network operator.
The company has been recording its support calls for quality analysis and converting the same into a text form. Later it would run those texts through its advanced machine learning algorithms to find out the customers who are most distressed and were negatively affected by the last call they had with the company’s customer representative.
After identifying such individuals, the company would send them apologies in text messages with custom discounts. As a result, they have now automated customer retention mechanisms and have improved their churn rates.
Banking and Finance
With the help of AI-powered sentiment analysis, finance institutes, including banks, can improve their customer acquisition and retention strategies by investing in sentiment analysis technologies. They can gauge how customers are responding to the offers being run by their competitors on social media.
If the customers show positive sentiments on those campaigns, they can also consider framing something similar for their brand. The organizations can also learn about the customers’ major pain points with surgical precision.
In a sentiment analytics study conducted by a banking institution, they were able to pinpoint the major concern faced by most customers, which is the unavailability of support services during their free time, mostly during lunch hours. That helped the company invest its resources in the right direction, improving brand positioning and customer retention rates.
Effective Crisis Management
One of the very popular sentiment analysis use cases is crisis management. Sentiment analysis applications enable you to swiftly identify and address negative brand sentiment during challenging situations. By monitoring sentiment across various channels such as social media, news articles, and customer feedback platforms, you can detect early warning signs of potential crises and take proactive measures to mitigate reputational damage.
The sentiment analysis application offers you valuable insights into public sentiment, allowing you to craft timely and effective responses that resonate with your target audience. By leveraging AI sentiment analysis for crisis management, you can safeguard your brand reputation, maintain customer trust, and emerge stronger from adversity.
Market Research
Another most common use case of sentiment analysis is market research. In today’s competitive business landscape, market research is essential for staying ahead of the curve and identifying emerging trends and consumer preferences. However, doing all that work manually can result in delays and errors. That’s exactly where this application of sentiment analysis comes into play!
Sentiment analysis provides you with valuable insights into public opinion and sentiment surrounding your products, services, and industry trends. By analyzing sentiment data from different channels, you can gain a deeper understanding of consumer sentiment, identify market opportunities, and inform strategic decision-making.
Whether it’s launching a new product, entering a new market, or refining marketing strategies, sentiment analysis empowers you to make data-driven decisions that drive growth and success.
Social Media Monitoring
Social media has become a powerful platform for brand communication and customer engagement, making it essential for businesses to monitor and manage their online presence effectively. However, you cannot track every comment or every mention that happened on various social media platforms for your brand. That’s where this use case of sentiment analysis is beneficial!
Sentiment analysis enables you to track brand mentions, sentiment trends, and customer feedback across social media platforms in real-time. Now this crucial data can be used in two ways. One, you can identify positive brand sentiment and capitalize on opportunities to amplify positive buzz. On the other hand, you can detect negative sentiment early on to address customer concerns, resolve issues, and mitigate potential crises before they escalate.
By leveraging this sentiment analysis application for social media monitoring, you can enhance brand reputation, foster customer loyalty, and drive engagement.
Personalized Customer Interactions
In today’s hyper-connected world, delivering personalized customer experiences is key to building lasting relationships and driving customer loyalty. This specific use case of sentiment analysis enables you to tailor customer interactions based on individual preferences, sentiment, and feedback. By analyzing sentiment data from interactions, you can identify customer sentiment in real-time and adjust your communication strategies accordingly.
Whether it’s recommending products, resolving customer complaints, or delivering targeted marketing messages, sentiment analysis empowers you to deliver personalized experiences that resonate with your customers. By partnering with a trusted AI development company, you can easily leverage this use case of sentiment analysis to increase customer satisfaction, drive repeat purchases, and strengthen brand loyalty.
Fraud Detection
Fraudulent activities pose a significant threat to businesses across industries, resulting in financial losses, reputational damage, and legal consequences. We are sure you would be seeking proven fraud detection methods for your business. Enters AI sentiment analysis!
Sentiment analysis application offers a powerful tool for detecting potential fraud by analyzing sentiment patterns in textual data such as customer communications, reviews, and transaction records. By detecting anomalies and deviations from normal sentiment patterns, you can flag potentially fraudulent activities for further investigation.
Whether it’s identifying suspicious transactions, detecting fake reviews, or uncovering insider threats, AI sentiment analysis empowers you to mitigate fraud risks and protect your assets.
Public Relations & Influencer Marketing
Public relations and influencer marketing play a crucial role in shaping brand perception and driving consumer engagement in today’s digital age. But as a PR, how would you know the best influencer for marketing your brand on a global platform? That’s exactly where this application of sentiment analysis comes into play!
Sentiment analysis enables you to monitor public sentiment towards your brand, key influencers, and industry trends in real-time. By analyzing sentiment data from news articles, social media, and online discussions, you can forge strategic partnerships with influencers that resonate with your target audience and elevate your brand’s image. Similarly, by detecting negative sentiment early on, you can address potential issues, manage crises, and protect your brand reputation.
Customer Feedback Analysis
Customers are the king in today’s digital world; they can make or break your company’s reputation via reviews and feedback. In fact, customer feedback is a valuable source of insights if you are looking to improve products, services, and overall customer experience. Sentiment analysis application enables you to analyze customer feedback from various sources such as surveys, reviews, and social media mentions to gain actionable insights into customer sentiment and preferences.
This AI technology classifies the feedback into positive, neutral, and negative sentiments, to help you identify common pain points, areas for improvement, and emerging trends. Whether it’s enhancing product features, addressing customer complaints, or refining marketing strategies, sentiment analysis empowers you to make data-driven decisions that drive customer satisfaction and loyalty.
Brand Monitoring
Brand reputation is a cornerstone of business success, influencing customer trust, loyalty, and purchasing decisions. Sentiment analysis enables you to monitor online conversations about your brand, products, and industry in real-time.
By analyzing sentiment data from social media, news articles, and online reviews, you can gain valuable insights into brand sentiment trends, identify emerging issues, and respond proactively to customer feedback.
Whether it’s addressing negative reviews, amplifying positive sentiment, or managing brand crises, sentiment analysis application empowers you to protect and enhance your brand reputation. You can hire dedicated developers to create a sentiment analysis application for brand monitoring with which you can build trust with your audience, foster brand advocacy, and drive long-term growth.
Risk Management
As a business owner, your main goal would always be to safeguard your company assets, protect your brand reputation, and ensure long-term sustainability. But how can you mitigate the risks that do not facilitate your business goals? This use case of sentiment analysis is beneficial for you!
Sentiment analysis application enables you to monitor sentiment trends in real-time across various channels such as news articles, social media, and online discussions to identify potential risks and threats proactively.
With AI sentiment analysis, you can categorize the data into positive, neutral, and negative sentiments to assess trends, detect emerging risks, and take preventive measures to mitigate their impact.
Whether it’s managing brand crises, addressing negative publicity, or identifying market risks, sentiment analysis empowers you to stay ahead of potential threats and protect your interests effectively. By leveraging sentiment analysis for risk management, you can enhance your resilience, minimize financial losses, and maintain stakeholder trust in today’s dynamic business environment.
Employee Sentiment Analysis
If you are in the corporate sector, you might know the importance of employee engagement in running a successful business. Employee morale and engagement play a crucial role in driving productivity, performance, and organizational success. However, tracking their exact needs and sentiments is a tedious task, especially if done manually. That’s where this sentiment analysis application comes into play!
Sentiment analysis enables you to monitor employee sentiment, analyze feedback, and identify areas for improvement in real time. You can leverage real-time employee data from feedback forms, reviews, or chat conversations with HR to categorize this data into positive, neutral, and negative sentiments. With this sentiment data, you can assess employee satisfaction, identify key drivers of engagement, and address concerns proactively.
Whether it’s improving workplace culture, enhancing communication, or implementing targeted interventions, sentiment AI empowers you to create a positive work environment where employees feel valued, motivated, and empowered to succeed.
How Can Matellio Help You with AI Sentiment Analysis Application Development?
As a leading consultancy in the USA, we are focused on transforming enterprises using cutting-edge technologies and years-long expertise in digital transformation. Whether you need to implement new technology in your business or modernize your existing infrastructure, we have got the perfect resources and tools to cater to all your business requirements.
Here are some perks that you can enjoy by partnering with Matellio for sentiment analysis application development:
Expertise in AI and NLP
Our seasoned professionals are well-versed in machine learning consulting, NLP, and other AI development services. Leveraging our expertise, we tailor sentiment analysis models to your unique business needs, ensuring accurate results and actionable insights.
Customized Solutions
We understand that every business has distinct requirements. Matellio crafts bespoke sentiment analysis solutions, tailored to your industry, target audience, and objectives. Whether it’s social media sentiment analysis or customer feedback interpretation, our custom enterprise solution development services align with your goals.
Advanced Tools and Technologies
Our arsenal includes cutting-edge tools and technologies that drive sentiment analysis to new heights. From machine learning algorithms to deep learning frameworks, we deploy the latest innovations, ensuring superior performance and precision in sentiment analysis.
Scalable Infrastructure
Matellio provides scalable infrastructure solutions, using cloud integration services, accommodating your evolving data needs. Whether you’re processing small datasets or analyzing vast volumes of information in real-time, our infrastructure scales seamlessly, guaranteeing uninterrupted sentiment analysis operations.
Integration Support
Seamless integration is pivotal for successful sentiment analysis implementation. Matellio offers comprehensive integration support, ensuring smooth alignment with your existing systems and workflows. Whether it’s API integration or system compatibility, we streamline the process, minimizing disruptions and maximizing efficiency.
Ongoing Support and Maintenance
Our commitment doesn’t end with implementation. Matellio provides ongoing support and maintenance services, ensuring your sentiment analysis solution remains robust and effective in the long run. From troubleshooting issues to optimizing performance, and even legacy software modernization services, we’re with you every step of the way.
With Matellio as your trusted partner, implementing sentiment analysis becomes a streamlined and rewarding journey, unlocking valuable insights and driving informed decision-making across your organization.
Conclusion
To conclude, we can say that in today’s digital age, the customer reigns supreme. Their voices, expressed through online reviews, social media comments, and surveys, hold a wealth of valuable insights. Sentiment AI is the technology you need to decipher the emotions behind this customer data. By leveraging its power, you can unlock a treasure trove of customer insights, transforming raw data into actionable intelligence.
The applications of sentiment analysis go beyond just measuring positive or negative feedback. It empowers you to truly understand customer sentiment, identify trends, address pain points, and optimize your business strategies for success.
Ready to unlock the power of customer voice? Investing in sentiment analysis application development is a strategic move that can propel your business forward, placing you at the forefront of customer-centricity in 2024 and beyond. Hence, partner with a trusted consultancy who can deliver tailored technology consulting services to develop a custom sentiment analysis solution that aligns with your specific needs and unlocks a world of customer insights.
Sentiment Analysis Development – FAQ’s
Q1. What is the use of sentiment analysis?
Sentiment analysis, also known as opinion mining, is used to analyze the sentiment, opinion, or emotion expressed in textual data. Its primary purpose is to understand the subjective information conveyed in text and extract insights to inform decision-making processes. Some common uses include:
- Brand monitoring and reputation management
- Customer feedback analysis
- Market research and competitor analysis
- Social media monitoring
- Product and service improvement
- Fraud detection
- Risk management
- Public relations and crisis management
Q2. How much does it cost to develop a sentiment analysis application?
The cost of developing a sentiment analysis application can vary depending on several factors, including the complexity of the project, the scale of implementation, the technology stack used, and the level of customization required. You can schedule a free 30-minute call with our experts to discuss your requirements and get a no-obligation quote for your project!
Q3. How can we get started with Matellio for sentiment AI application development?
Reaching out to Matellio for sentiment analysis application development is a simple process:
- Fill out our form for a free 30-minute consultation
- Discuss your ideas and requirements with our experts
- Our consultants will analyze your needs and propose a custom solution
- Choose a suitable engagement model
- Begin your project
Q4. Which companies use sentiment analysis?
Sentiment analysis is widely used across various industries by companies of all sizes. Some notable examples of companies using sentiment analysis include Facebook, Twitter, Instagram, Amazon, eBay, Netflix, etc.
Q5. What resources do I need to implement AI sentiment analysis in my business?
Implementing AI sentiment analysis in your business requires several key resources:
- Data Scientists
- Machine Learning Engineers
- Data Engineers
- Software Developers
- UI/UX Designers
- Testers
- DevOps Team
- Project Manager