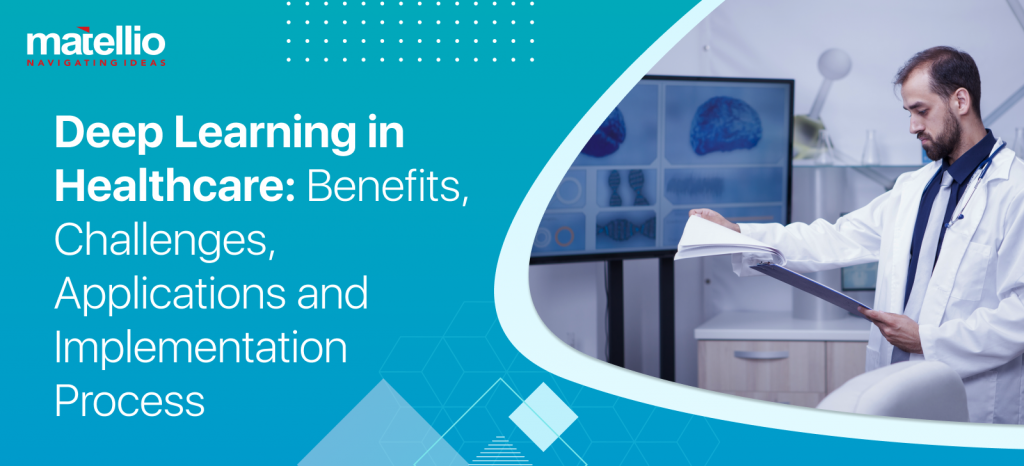
The healthcare industry is undergoing a transformation fueled by next-generation technologies that are reshaping the way diseases are diagnosed, treated, and managed. With advancements in various next-gen technologies, your business catering to healthcare can leverage cutting-edge tools and techniques to deliver more personalized, efficient, and effective care to patients.
Amid these technologies, deep learning in healthcare stands out as a powerful force innovating and revolutionizing the entire healthcare ecosystem.
From medical imaging and diagnostics to clinical decision support and predictive analytics, deep learning is transforming every aspect of healthcare delivery, empowering clinicians with powerful tools to make more informed decisions and improve patient outcomes.
So, if you are trying to invest in implementing the power of deep learning within your healthcare business, it can do wonders! The blog here is designed to showcase some of the vital aspects of this digital asset that you should explore before making your decision.
Let’s get started!
Market Stats,
Perks of Implementing Deep Learning in Healthcare
Investing in enterprise solutions development driven by deep learning can upgrade your business catering to the healthcare sector. It offers compelling reasons for businesses looking to drive innovation, improve patient care, and capture value in a rapidly evolving industry landscape. Here’s a detailed exploration of why your business should consider choosing deep learning:
Transformative Potential
The first perk of choosing deep learning for your business catering to healthcare is that it can unlock insights from vast amounts of complex data that were previously inaccessible or difficult to interpret. By leveraging advanced algorithms and neural networks, deep learning can uncover patterns, correlations, and predictive insights.
Enhanced Diagnostic Accuracy
Deep learning algorithms have demonstrated remarkable accuracy in interpreting different medical images such as X-rays, MRI scans, and pathology slides. By augmenting the capabilities of healthcare providers, deep learning can improve diagnostic accuracy, reduce errors, and expedite the detection of critical conditions such as cancer, neurological disorders, and cardiovascular diseases.
Efficient Clinical Workflows
Deep learning in healthcare can streamline and optimize clinical workflows by automating repetitive tasks, flagging abnormalities for further review, and prioritizing cases based on urgency and complexity. By integrating deep learning solutions into electronic health record (EHR) systems and radiology platforms, healthcare providers can enhance efficiency, reduce administrative burdens, and allocate resources more effectively.
Personalized Medicine
The digital asset called deep learning enables the analysis of diverse datasets. These datasets include genomic profiles, clinical histories, and lifestyle factors, and can generate personalized treatment recommendations tailored to individual patients. Using the ML modles and predictive analytics, deep learning can predict treatment responses, identify optimal therapies, and sort patients based on their risk profiles.
Accelerated Drug Discovery and Development
Applications of deep learning in healthcare can expedite the drug discovery and development process by analyzing molecular structures, biological pathways, and drug interactions at an unprecedented scale and speed.
By identifying promising drug candidates, predicting potential targets, and optimizing clinical trial designs, deep learning can shorten development timelines, reduce costs, and increase the success rates of new therapeutics, ultimately bringing life-saving treatments to market faster.
Remote Monitoring and Telemedicine
Deep learning enables the analysis of real-time data streams from wearable devices, remote monitoring systems, and telemedicine platforms, facilitating proactive health management and timely interventions outside traditional healthcare settings. By monitoring vital signs, detecting anomalies, and predicting exacerbations, deep learning can empower patients to take control of their health.
Competitive Advantage and Market Differentiation
Investing in deep learning in healthcare can provide your business with a significant competitive advantage and differentiate your offerings in a crowded marketplace. By delivering innovative solutions that improve patient outcomes, enhance clinical workflows, and drive operational efficiencies, your business can position itself as a leader in the rapidly evolving healthcare landscape.
Ethical Imperatives and Social Impact
Beyond the potential for financial returns, investing in deep learning in healthcare aligns with ethical imperatives and social impact goals. By advancing the frontiers of medical science, democratizing access to healthcare, and addressing unmet clinical needs, your business can make a meaningful contribution to society.
Want to Integrate the Power of Deep Learning Within Different Processes of Healthcare?
How Can AI Enhance the Integration of Deep Learning in Healthcare?
Artificial Intelligence (AI) can play a crucial role in enhancing the integration of deep learning by providing complementary tools, techniques, and infrastructure to support the development, deployment, and utilization of deep learning solutions.
You can leverage the best digital transformation services and can get the guidance of experts to understand how to use the power of AI for upgrading your current business approach. Here are several ways in which AI can enhance the integration of deep learning within different processes of your healthcare sector:
Data Processing and Management
AI techniques, such as natural language processing (NLP) and data mining, can be used to preprocess and extract structured information from unstructured healthcare data sources, such as clinical notes, medical images, and biomedical literature. By automating data processing tasks, AI can facilitate the integration of diverse data types into deep learning pipelines, enabling more comprehensive and holistic analyses of healthcare data.
Feature Engineering and Selection
AI algorithms can assist in feature engineering and selection by identifying relevant features and representations from raw data that are most informative for deep learning models. By leveraging expert guidance from a reputed AI development company, you can learn about different techniques to streamline the model development process. These techniques can include dimensionality reduction, feature selection, and autoencoder-based feature learning.
Model Development and Optimization
AI frameworks and platforms, such as TensorFlow, PyTorch, and Keras, provide robust tools and infrastructure for developing, training, and optimizing deep learning models. By leveraging AI platforms, researchers and developers can experiment with different architectures, hyperparameters, and optimization techniques to improve the performance and generalization of deep learning models in healthcare tasks.
Clinical Decision Support Systems (CDSS)
AI-driven CDSS can integrate deep learning models into clinical workflows to assist healthcare providers in making informed decisions about patient care. By leveraging patient data, medical knowledge bases, and evidence-based guidelines, AI-powered CDSS can provide real-time recommendations, alerts, and predictions to support diagnosis, treatment planning, and disease management in clinical settings.
Healthcare Analytics and Insights
AI techniques, such as ML and predictive analytics, can analyze large-scale healthcare datasets to uncover patterns, trends, and insights that inform decision-making and improve healthcare delivery. By integrating deep learning models into analytics platforms, AI can enable more accurate predictions, risk stratifications, and outcome assessments, facilitating data-driven decision-making and quality improvement initiatives in healthcare organizations.
Clinical Imaging and Diagnostics
AI-driven imaging analytics can leverage deep learning models to analyze medical images and assist radiologists and clinicians in diagnosing diseases, detecting abnormalities, and quantifying disease progression. By integrating deep learning algorithms into imaging platforms and Picture Archiving and Communication Systems (PACS), AI and deep learning in healthcare can improve the efficiency, accuracy, and consistency of diagnostic interpretations in radiology and pathology departments.
Also Read- How to Develop a Medical Imaging Analysis Software Solutions?
Personalized Medicine and Precision Treatment
AI-powered personalized medicine platforms can leverage deep learning models to analyze multi-modal patient data and generate tailored treatment recommendations based on individual characteristics, preferences, and clinical profiles. By integrating deep learning algorithms into electronic health record (EHR) systems and clinical decision support tools, AI can enable precision medicine approaches that optimize treatment outcomes and improve patient satisfaction and adherence.
Regulatory Compliance and Quality Assurance
AI-driven solutions can support regulatory compliance and quality assurance efforts by automating tasks such as data validation, documentation, and reporting. By integrating deep learning models into compliance management systems and quality control processes, AI can ensure adherence to industry standards, regulatory requirements, and best practices in healthcare delivery, enhancing patient safety and data integrity.
Addressing Challenges with Deep Learning
Traditionally, different healthcare operations cannot function seamlessly due to some challenges. However, integrating deep learning in healthcare can help address several traditional challenges that your business might face in the healthcare industry. Here are some of these challenges and how deep learning can offer solutions:
Diagnostic Accuracy and Efficiency
- Challenge: Traditional diagnostic methods may be prone to errors or inconsistencies due to human subjectivity, variability, and fatigue.
- Solution: Deep learning algorithms can assist healthcare providers in interpreting medical images, analyzing clinical data, and making accurate diagnoses. By automating repetitive tasks and flagging abnormalities, deep learning can enhance diagnostic accuracy, improve efficiency, and reduce the risk of diagnostic errors.
Personalized Medicine and Precision Treatment
- Challenge: Traditional approaches to healthcare may adopt a one-size-fits-all approach, overlooking individual variability in patients’ genetic makeup, physiological characteristics, and treatment responses.
- Solution: Deep learning enables the analysis of multi-modal data, including genomic profiles, clinical histories, and lifestyle factors, to generate personalized treatment recommendations tailored to individual patients. By developing deep learning solutions for personalized medicine, businesses can empower healthcare providers to deliver targeted interventions, optimize treatment outcomes, and improve patient satisfaction and adherence.
Drug Discovery and Development Costs and Timelines
- Challenge: The drug discovery and development process are lengthy, costly, and associated with high rates of failure. Traditional methods may involve trial-and-error approaches and labor-intensive experiments that are time-consuming and resource-intensive.
- Solution: Custom building the solutions driven by AI and deep learning in healthcare can accelerate the drug discovery and development process by analyzing molecular structures, biological pathways, and drug interactions to identify promising drug candidates and predict their efficacy and safety profiles.
By leveraging deep learning algorithms for virtual screening, molecular docking, and predictive modeling, businesses can expedite the identification of potential therapeutics, reduce development timelines, and lower costs, ultimately bringing life-saving treatments to market faster.
Regulatory Compliance and Quality Assurance
- Challenge: Healthcare organizations must adhere to stringent regulatory requirements and quality standards to ensure patient safety, data privacy, and ethical conduct. Traditional approaches to regulatory compliance and quality assurance may be manual, time-consuming, and error prone.
- Solution: Deep learning can support regulatory compliance and quality assurance efforts by automating tasks such as data validation, anomaly detection, and regulatory reporting. By developing deep learning solutions for regulatory compliance, businesses can streamline processes, reduce administrative burdens, and mitigate risks, ensuring adherence to industry standards and regulations while maintaining high standards of patient care and safety.
Beat the Traditional Challenges by Implementing Deep Learning Solution!
Applications of Deep Learning in Healthcare
There is a wide range of deep learning use cases across different industries; here, we have mentioned some of the vital applications of this advanced technology in the healthcare sector:
Medical Imaging Analysis
- Detection of Diabetic Retinopathy: Deep learning models can analyze retinal images to detect signs of diabetic retinopathy, a leading cause of blindness. By identifying lesions, microaneurysms, and hemorrhages, deep learning algorithms can assist ophthalmologists in early diagnosis and intervention.
- Brain Tumor Segmentation: Deep learning techniques can segment MRI scans to delineate brain tumors and surrounding tissues, aiding neurosurgeons in treatment planning and tumor resection while minimizing damage to healthy brain tissue.
Clinical Decision Support Systems (CDSS)
- Early Sepsis Detection: Deep learning algorithms can analyze vital signs, laboratory results, and clinical notes from electronic health records (EHRs) to predict the onset of sepsis in hospitalized patients.
- Drug Interaction Prediction: Another deep learning application in healthcare is that its models can predict potential drug-drug interactions based on patients’ medication histories, genetic profiles, and clinical parameters. By providing clinicians with real-time alerts and recommendations, CDSS can prevent adverse drug reactions and enhance medication safety.
Drug Discovery and Development
- Molecular Docking and Drug Design: Deep learning in healthcare can accelerate the process of molecular docking and virtual screening to identify potential drug candidates and predict their binding affinities to target proteins. By simulating molecular interactions and predicting drug-receptor interactions, deep learning algorithms can expedite the discovery of novel therapeutics for various diseases.
- Drug Repurposing: Deep learning models can analyze large-scale omics data to identify existing drugs that may be repurposed for new indications. By uncovering unexpected connections between drugs and diseases, drug repurposing can shorten development timelines, reduce costs, and bring treatments to market faster.
Personalized Medicine
- Genomic Risk Stratification: Deep learning algorithms can analyze genomic data to identify genetic variants associated with disease risk, treatment response, and drug metabolism. By integrating genomic information with clinical data, personalized medicine can tailor treatment plans to individual patients’ genetic profiles, optimizing therapeutic outcomes and minimizing adverse effects.
- Precision Oncology: Deep learning in healthcare can analyze multi-omics data, including genomics, transcriptomics, and proteomics, to identify actionable mutations and molecular subtypes in cancer patients. By guiding targeted therapy selection and patient stratification, precision oncology can improve survival rates and quality of life for cancer patients.
How To Implement Deep Learning in Healthcare?
Implementing deep learning in healthcare to enhance your business involves strategic planning and execution. Here are some strategies or ways to effectively implement this advancement in healthcare to enhance the entire workflow of your business:
Identify Priority Areas
Assess your business goals, challenges, and opportunities in healthcare. Identify priority areas where deep learning can add value, such as medical imaging analysis, clinical decision support, predictive analytics, or personalized medicine.
Build Expertise
Invest in building expertise in deep learning within your organization. Hiring or training data scientists, machine learning engineers, can be time consuming and can be heavy on your budget. So, we would suggest you hire domain experts with knowledge of healthcare to lead deep learning initiatives. You can leverage the business consulting services from reputable companies like Matellio and get yourself a team of experts.
Data Collection and Annotation
The team you choose should help you in gathering high-quality, labeled datasets for training deep learning models. The experts in your team can offer you access to diverse and representative datasets, including medical images, electronic health records (EHRs), genomic data, and clinical notes. Invest in data annotation and curation to ensure data quality and consistency.
Select Appropriate Architectures
When implementing deep learning in healthcare, you must choose architectures and algorithms that are well-suited to your use cases and data types. Experiment with different architectures and model configurations to find the most effective solutions.
Train and Validate Models
The experts can train deep learning models using labeled data, adjusting hyperparameters and optimization techniques as needed. Validate the trained models using appropriate evaluation metrics and validation techniques to ensure robustness and generalization to unseen data. Consider using techniques such as cross-validation and data augmentation to improve model performance and mitigate overfitting.
Integration with Healthcare Systems
Integrate the trained deep learning models into existing healthcare systems or develop standalone applications as needed. Ensure compatibility with healthcare IT infrastructure and provide APIs or SDKs for seamless integration with third-party applications and workflows.
Regulatory Compliance and Ethical Considerations
It is vital to ensure compliance with regulatory requirements, such as HIPAA, GDPR, and FDA regulations, to protect patient privacy and data security. Consider the ethical implications of deep learning applications in healthcare, including bias mitigation, fairness, transparency, and accountability. Document model development and validation processes to demonstrate compliance with regulatory standards and best practices.
Consult Experts and Explore the Process of Implementing Deep Learning Within Your Business!
How Can Matellio Help with the Implementation of Deep Learning in Healthcare?
After scrolling through the entire blog and gaining clarity about different aspects of implementing deep learning in healthcare, you can now understand how fruitful this technological advancement can be for your business. However, partnering with the right team to guide you in this next-gen asset is very important.
This is where you can trust Matellio!
- We specialize in developing custom deep-learning solutions tailored to specific healthcare needs. Whether it’s medical image analysis, clinical decision support, or predictive analytics, Matellio can design and develop deep learning models to address your unique requirements.
- Our experts have experience working with next-gen technologies, and they can offer you AI, IoT, deep learning, and machine learning consulting services so that you understand how you can align these advanced technologies to sustain your business growth.
- Matellio can seamlessly integrate deep learning solutions into existing healthcare systems and workflows, ensuring compatibility with electronic health record (EHR) systems, medical imaging platforms, and clinical decision support systems (CDSS).
- Our experts can develop scalable and cost-effective deep learning solutions that align with your budget and scalability requirements. Whether you need on-premises deployment, cloud-based solutions, or hybrid architectures, Matellio can design and implement solutions that meet your business needs while optimizing resource utilization and cost efficiency.
If you have any queries or wish to learn more about the implementation or integration process, timeline, or cost, our experts are here to assist you. Feel free to reach out to us through the provided form,
FAQ
What data sources and types do you utilize to train deep learning models in healthcare?
In healthcare, we leverage a wide range of data sources, including electronic health records (EHRs), medical imaging archives (such as X-rays, MRIs, and CT scans), genomic data, and real-time monitoring data from devices and sensors. By utilizing diverse data types, we ensure that our deep learning models are trained on comprehensive and representative datasets, enabling them to capture the complexity and variability of healthcare information.
How do you ensure adherence to regulatory requirements?
Compliance with regulatory requirements, such as HIPAA, GDPR, and other healthcare regulations, is paramount in our approach to implementing deep learning in healthcare. We implement stringent data security measures, privacy controls, and compliance frameworks to safeguard patient data and ensure adherence to regulatory standards throughout the deep learning implementation process.
How do you handle model maintenance, updates, and ongoing support post-implementation?
Our commitment to providing ongoing model maintenance, updates, and support post-implementation is integral to the success and sustainability of deep learning solutions in healthcare. We proactively monitor model performance, identify opportunities for optimization, and implement updates as needed to ensure the continued effectiveness and relevance of the solutions. Additionally, we provide guidance to users, enabling them to maximize the value and impact of deep learning in their healthcare operations.
What measures do you take to ensure the security and confidentiality of patient data?
Ensuring the security and confidentiality of patient data is a top priority in our approach to deep learning implementation in healthcare. We employ industry-leading encryption techniques, access controls, audit trails, and data anonymization methods to protect patient information from unauthorized access, disclosure, or misuse.
What are the expected timelines and costs associated with implementing deep learning in our healthcare business?
Timelines and costs for implementing deep learning in healthcare vary depending on factors such as project scope, complexity, and specific requirements. We provide transparent and detailed timelines and cost estimates tailored to each project, considering factors such as data acquisition, model development, testing, deployment, and ongoing support. Our goal is to deliver value-driven solutions that align with your budget and timeline constraints while maximizing the impact and effectiveness of deep learning in your healthcare business.