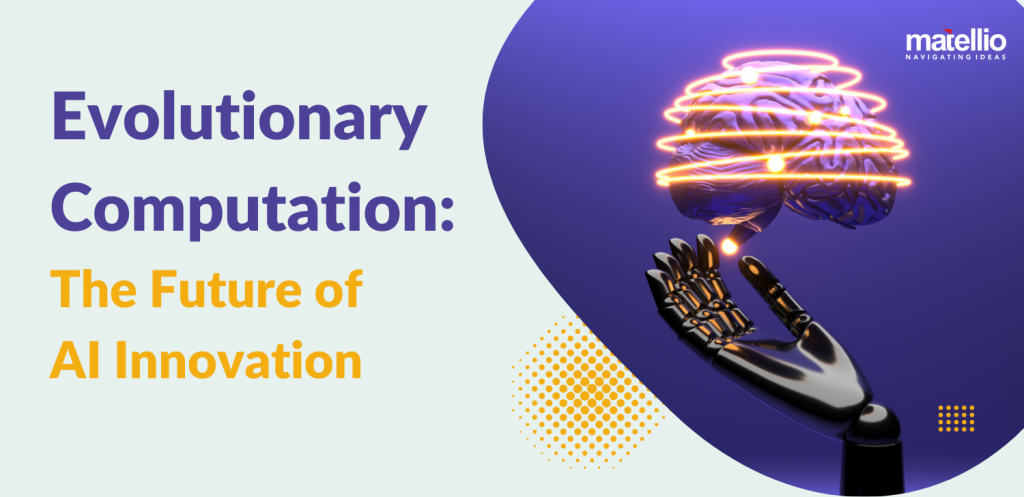
Introduction to Evolutionary Computation
In the dynamic world of Artificial Intelligence (AI), evolutionary computation is emerging as a groundbreaking force. This innovative approach, inspired by biological evolution, applies principles such as mutation, selection, and inheritance to solve complex computational problems. It stands out for its ability to evolve solutions to problems that are otherwise difficult for traditional AI methods to tackle.
The significance of evolutionary computation in AI is reflected in the market’s growth. According to Grand View Research, the global AI market, encompassing evolutionary computation, is projected to reach an astounding $1.81 trillion by 2030, growing at a CAGR of 37.3% from 2023. This surge underscores the increasing adoption and integration of evolutionary computation in diverse AI applications, marking it as a key driver in the evolution of AI technologies.
As a pioneering AI Development Company, we at Matellio recognize the transformative impact of evolutionary computation. We leverage these algorithms to develop solutions that are not only innovative but also adaptive and efficient, aligning with the evolving needs of modern industries.
Evolutionary Computation Use Cases
Transforming Industries with Advanced AI
Technology consulting services for Evolutionary computation are revolutionizing various sectors by offering unique approaches for business solutions. Here are ten impactful use cases.
Finance – Enhanced Trading Algorithms
In finance, evolutionary computation algorithms revolutionize trading strategies by evolving and adapting to market changes, resulting in more effective risk management and investment decision-making. This approach exemplifies how a Generative AI Services provider can offer cutting-edge solutions for the financial sector.
Healthcare – Drug Discovery and Personalized Medicine
In healthcare, these algorithms facilitate groundbreaking research in drug discovery and personalized treatment plans, adapting to individual genetic makeups for optimal results.
Aerospace – Design Optimization
Evolutionary computation is used to optimize the design of aircraft and spacecraft components, leading to safer and more efficient designs.
Automotive – Vehicle Routing and Scheduling
This technology optimizes routing and scheduling, enhancing efficiency in logistics and delivery services.
Energy – Smart Grid Management
In the energy sector, it’s used for managing smart grid systems, optimizing energy distribution and consumption.
Robotics – Adaptive Learning Systems
For robotics, evolutionary computation algorithms enable robots to learn and adapt to new environments, making them more autonomous and efficient.
Retail – Inventory Management
These algorithms optimize inventory levels, predicting consumer demand patterns, and reducing overstock or stockouts.
Telecommunications – Network Design and Optimization
Evolutionary computation helps in designing and optimizing network layouts, ensuring maximum coverage and efficiency.
Manufacturing – Process Optimization
It’s used to streamline manufacturing processes, enhancing productivity and reducing waste.
Also Read: AI Use Cases in Manufacturing.
Environmental Science – Ecosystem Modeling
These algorithms model complex ecosystems, helping in understanding and predicting environmental changes.
These use cases demonstrate the broad and impactful application of evolutionary computation across industries. They illustrate how evolutionary computation, especially when leveraged by an AI Development Company, can provide sophisticated solutions to complex problems. The next sections will delve deeper into the integration of evolutionary computation in AI systems, exploring its mechanics, algorithms, and future potential.
The Mechanics of Evolutionary Computation in AI
Enhancing AI with Evolutionary Strategies – Evolutionary computation brings a unique approach to AI problem-solving and system development. Its integration into AI systems is marked by several key characteristics:
Algorithmic Diversity and Evolution
- Evolutionary computation introduces a diverse array of algorithms, each with unique capabilities to solve specific problems.
- These algorithms evolve over time, continuously improving their efficiency and effectiveness in problem-solving.
Adaptive Problem-Solving
- AI systems equipped with evolutionary algorithms can adapt to changing environments and requirements, making them exceptionally versatile.
- This adaptability is crucial in fields like autonomous vehicles and adaptive traffic systems, where real-time decision-making is essential.
Robust Optimization Techniques
- In complex optimization problems, such as supply chain logistics or network configuration, evolutionary computation provides robust solutions that traditional algorithms might miss.
- These techniques excel in multi-objective optimization, balancing various factors to find the best possible solutions.
Innovative Design and Creativity
- Evolutionary computation can foster innovation and creativity in AI systems, particularly in fields like generative design in architecture or new material development.
- By exploring a vast space of possibilities, these algorithms can suggest solutions that are non-obvious and highly innovative.
Dynamic Learning and Improvement
- The iterative nature of evolutionary algorithms means that AI systems can learn from past experiences, making them more efficient over time.
- In scenarios like dynamic market analysis or evolving user preferences in digital marketing, this ability to learn and improve is invaluable.
Scalability and Flexibility
- Evolutionary computation algorithms are scalable, capable of handling problems of varying sizes and complexities.
- Their flexibility allows for application in diverse domains, from simple automation tasks to complex strategic planning in business environments.
Enhanced Data Analysis and Interpretation
- These algorithms can process and interpret vast amounts of data, providing insights that might be missed by human analysis.
- In areas like big data analytics and customer behavior analysis, this enhanced data interpretation capability is crucial.
Personalization and User Experience
- AI systems powered by evolutionary computation can tailor experiences and solutions to individual users, enhancing personalization in services and products.
- In sectors like e-commerce and personalized healthcare, this leads to improved user satisfaction and engagement.
Also Read: Role of AI in Cloud Computing
Exploring Evolutionary Computing
The Broad Spectrum of Evolutionary Computing
Evolutionary computing, a subset of evolutionary computation, encompasses a range of methodologies and applications. Its impact is far-reaching, influencing numerous fields with its unique approach to problem-solving.
Complex System Simulation and Modeling
Evolutionary computing is pivotal in simulating complex systems, such as ecological systems or financial markets, providing insights into their dynamics and potential future behaviors.
Optimization in Engineering and Design
In engineering, it’s utilized for optimizing designs, from aerodynamic shapes in automotive and aerospace to efficient layouts in civil engineering.
Enhancing Artificial Neural Networks
It plays a role in training and optimizing artificial neural networks, particularly in deep learning, by evolving network architectures or training algorithms for improved performance.
Game Development and Strategy Formulation
Evolutionary computing contributes to game development, particularly in creating complex, adaptive AI opponents or evolving game strategies.
Behavioral Modeling and Social Simulation
It’s used in behavioral modeling, simulating social phenomena or economic behaviors, providing valuable insights for policy-making and social sciences.
Bioinformatics and Medical Research
In bioinformatics, evolutionary computing aids in analyzing biological data, contributing to advancements in medical research and drug development.
Environmental Management and Conservation
It assists in environmental management, helping model and predict environmental changes, crucial for conservation efforts and policy development.
Advanced Robotics and Autonomous Systems
Evolutionary computing is key in developing advanced robotics, particularly in evolving autonomous systems capable of complex tasks and decision-making.
Data Mining and Knowledge Discovery
It enhances data mining processes, extracting meaningful patterns and insights from large datasets, crucial in big data applications.
Creative Industries and Art
In creative industries, it’s used for generative art and music, where algorithms create novel and unique artistic expressions.
Also Read: Enterprise AI: Driving the Business in Future.
Evolutionary Computation Algorithms
The Building Blocks of Advanced AI
The algorithms used in evolutionary computation are diverse, each offering unique advantages for various applications in AI.
Genetic Algorithms (GAs)
Principle: Mimic natural selection and genetics. They work by evolving solutions to problems using a population of candidate solutions.
Applications: Used extensively in optimization problems, machine learning parameter tuning, and evolving solutions in dynamic environments.
Genetic Programming (GP)
Principle: Involves evolving computer programs or expressions. GP uses tree structures to represent these programs.
Applications: Automating the design of algorithms, symbolic regression, and evolving models for predictive analytics.
Evolutionary Strategies (ES)
Principle: Focus on evolving strategies or rulesets. They emphasize mutation over crossover.
Applications: Applied in continuous optimization problems and adaptive control systems.
Differential Evolution (DE)
Principle: A population-based algorithm that uses differential changes to evolve solutions.
Applications: Effective in multi-modal optimization problems and engineering design optimization.
Particle Swarm Optimization (PSO)
Principle: Inspired by social behavior patterns, PSO optimizes a problem by iteratively trying to improve a candidate solution.
Applications: Widely used in neural network training and data clustering.
Ant Colony Optimization (ACO)
Principle: Inspired by the foraging behavior of ants and their ability to find shortest paths.
Applications: Effective in discrete optimization, routing problems, and scheduling tasks.
Evolutionary Multi-objective Optimization (EMO)
Principle: Focuses on optimizing several objectives simultaneously, often involving trade-offs between objectives.
Applications: Used in areas requiring a balance of multiple objectives, like sustainable engineering and resource management.
Coevolutionary Algorithms
Principle: Involve multiple evolving populations that interact, mimicking an ecosystem.
Applications: Useful in game theory, competitive environments, and modeling complex adaptive systems.
Also Read: Machine Learning as a Service (MLaaS).
Conclusion: Embracing Evolutionary Computation for AI Advancement
Evolutionary computation represents a significant leap in AI development, offering enterprise solutions that are adaptive, efficient, and innovative. The key takeaways from this exploration include.
- Versatility in Application: Its use across various industries, from healthcare to finance, demonstrates its adaptability and wide-ranging impact.
- Innovative Problem-Solving: Evolutionary algorithms excel in tackling complex problems that traditional AI methods find challenging.
- Continuous Evolution and Learning: These algorithms ensure that AI systems are not static but evolve to become more efficient and effective over time.
- Cross-Disciplinary Potential: The principles of evolutionary computation are applicable across multiple disciplines, fostering innovation and creativity.
- Future Potential: As technology advances, the scope of evolutionary computation in AI will expand, leading to more sophisticated and nuanced applications.
As we look forward, the integration of evolutionary computation in AI is poised to drive significant advancements in technology. For businesses and industries looking to leverage these advancements, partnering with an expert like a Software Development Company specializing in AI can be a strategic move.
Final Thoughts
The journey into the world of evolutionary computation and AI is an exciting venture into the future of technology. For organizations aiming to stay at the forefront of innovation, exploring these technologies is not just an option but a necessity.
Ready to Explore Evolutionary Computation for Your AI Initiatives?
Contact Matellio – your digital transformation services provider for AI and evolutionary computation. Let’s shape the future together!
FAQs
What is evolutionary computation in AI?
Evolutionary computation in AI refers to a set of algorithms inspired by biological evolution. These algorithms simulate processes like mutation, selection, and inheritance to solve complex problems. They are particularly useful for optimization, evolving strategies, and adapting solutions in AI.
How does evolutionary computation differ from traditional AI methods?
Unlike traditional AI methods that often require explicit programming for problem-solving, evolutionary computation generates solutions through a process of evolution. This approach is dynamic, allowing algorithms to adapt and improve over time, which is especially effective in complex or changing environments.
Can evolutionary computation be applied in real-world scenarios?
Absolutely. Evolutionary computation has diverse real-world applications, including optimizing financial trading strategies, drug discovery in healthcare, improving logistics and supply chain management, and enhancing autonomous robotic systems.
What are some common algorithms used in evolutionary computation?
Key algorithms in evolutionary computation include Genetic Algorithms, Genetic Programming, Evolutionary Strategies, and Particle Swarm Optimization. Each of these algorithms has unique characteristics and is suited for different types of problems and applications.
What is the future of evolutionary computation in AI?
The future of evolutionary computation in AI is promising, with potential for further advancements in areas like adaptive learning systems, complex problem-solving, and integrations with other AI technologies. It's expected to play a critical role in developing more sophisticated, efficient, and adaptable AI systems.