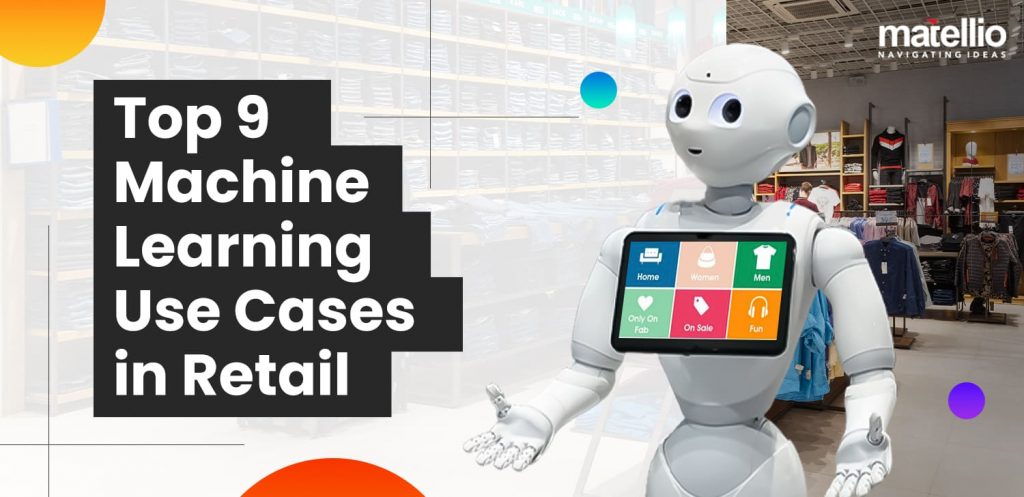
Machine learning tops as the most promising technologies of the decade, and the retail industry finds its uses in many places. The technology isn’t exclusive to big enterprises anymore, and many SMBs are investing in the space, reaping benefits. Whether one uses it for management, operations, or sales, machine learning can impact either majorly. A good plan is a must before diving into it, or else the chances of failure skyrocket.
If you are already versed in the possibilities, that’s great. If not, we are here to help you. Today’s blog covers essentials, including why retail needs machine learning, the top nine machine learning use cases in retail, and the major players using it. Like always, you have the option to opt for a free consultation when you reach out to us. As a machine learning consulting company, it will be our pleasure to assist you with any questions you have in the regard.
Why Does Retail Need Machine Learning?
Let’s begin with the whys first. A small to medium successful retail business wouldn’t benefit from ML implementation, is what most people think. So, why exactly does retail need machine learning? The market is changing rapidly, and while offline and online co-exist, there are other major changes required by business owners to keep up. Machine learning applications in retail include generating actionable data for almost all processes there are.
Without it, you would be wasting many opportunities, and you would do that while others use it to their full potential. From inventory management to demand forecasting, there are major use cases of ML in retail, and we will discuss those below. We are not asking you to invest without thinking things through, but a consultation with a machine learning consulting company will help you see through.
Top Nine Use Cases of Machine Learning in Retail
Dynamic Pricing
The algorithm is backed by different facts generated with different methodologies. There are three classifications for the same, which uses machine learning as a primary source for price determination.
Bayesian Model
It works on Baye’s theorem, through which price can be determined based on a variety of data collected from the past. The factors that would weigh in depend on how deep you want to implement, but it usually can only work around a factor or two.
Reinforcement Model
The reinforcement model changes parameters based on collected data and compares it with one or more present parameters. The model is goal oriented and is focused on collecting and using a user’s environment’s data.
Decision Tree Model
This model adds a layer where weighted decisions can overpower and be combined to make a better decision. As a simple example, it being summer season would overpower other factors to a ratio to reduce the price of winter apparel for the time being.
Product Placement
There are tons of variables affecting product placement in a retail environment. The simpler manual way to do it goes through each item’s sales and correlates it with their placement across the store. Though simple, it would be impossible to do so for thousands of products and various brands of those to choose from.
The point to be noted here is that the decision tree required inputs for both product type and brand, making it twice as tough. Machine learning is implemented to experiment and gather data which could help decide the future possibilities of product placement.
The strategy is different for each type of commodity a store sells and overlaps with broader categories such as clothing, electronics, FMCG, etc. The algorithm will help you determine the good most sold based on marked positions, and you can keep experimenting till you reach satisfactory sales for each product.
Recommendation Engine
According to a McKinsey study, over 35% of sales on Amazon are generated through their proprietary recommendation algorithm. We are talking about the biggest eCommerce platform in the world, and those numbers would baffle anyone. Well, that’s how important recommendation engines are, and you need machine learning to create one for yourself. There are three popular types of recommendation systems.
Collaborative Filtering
A collaborative filtering system works on data generated by other users. The recommender system focuses on what the other customers bought with an item and recommends the same products to the ones making the purchase. Though simple, the systems are hard to implement when it comes to filtering based on specifics, and implementing it also becomes tough.
Content-Based Filtering
A content-based filtering system creates a specific profile for each user. The system draws in the preferences of a specific user and maps them to other products to recommend them later. Various correlations are drawn to decide which customer would like what type of product, and the same can be implemented for such systems.
Hybrid Recommendation
A hybrid system is a combination of both of the above. The system generates a list of preferences generated by each collaborative system and content-based system. It then processes both to attain a list where items overlap and create a recommendation that is much more likely to be bought by the user purchasing a specific product.
Sales Prediction
Knowing what’s going to happen with your sales can be used as a superpower by a retail business. That’s why companies hire machine learning developers to create custom solutions that help analyze past data and make sales predictions for each product in your lineup. There can be multiple factors and ways to determine it based on your product types and business size.
Sales prediction can be as simple as determining it from past data charts and as complex as determining outliers and deviations to predict a much more accurate outcome. Machine learning steps in when data is enormous, and manual filtering and correlation are next to impossible or simply inefficient.
One must first gather the data generated over the years to gain a perspective of sales possibilities. The flip side of it is demand forecasting, which is an approach in the other direction and one we will discuss further in the blog. If you don’t have data with you and are starting new, ask your development company to make arrangements for gathering data in your retail software for the future.
Fraud Detection
A more secure retail environment can be created using a combination of computer vision services and machine learning. The major types of fraud in retail include:
- Transaction Fraud
- Triangulation Fraud
- Friendly Fraud
- Return Fraud
- Chargeback Fraud
An effective solution can be mapping users with their other social accounts and introducing 2FA. Machine learning algorithms can very well do fraud detection for each type of fraud. While there is no way to know if a transaction is fraudulent or not, there can be an introduction of ways to know if it is. Store and site owners can leverage AI to determine fraud and eliminate it or at least protect the users from it.
The goal of fraud prevention is drawing out patterns, and mapping detected frauds to secure the entire portal. Various companies already have an infrastructure in place to combat it, and you can get one developed by a retail software development partner in case you anticipate exposure with your site/store.
Targeted Marketing
Targeted marketing revolves around choosing a set of customers for campaigns who are more likely to make a purchase from an advertisement. Many factors play into consideration, making machine learning a must for a thorough implementation. The major benefits of targeted marketing include the following:
Better Recommendations
The model works toward generating better prospects for advertising and can work alongside the recommendation engine to determine relevant products.
Less Disturbance
Filter out disturbances to better settle products into one category than other. The same can also be used to make campaigns better suited for a product category than being focused on customers who will use them.
Reduced Costs
Marketing takes up a major budget for most companies, and targeted marketing through a machine learning algorithm can help save costs.
Increased Conversion
An increased conversion rate can be expected when relevant products are shown to people willing to make a purchase.
There are different approaches toward targeted marketing campaigns, and your machine learning consulting company will optimize investments based on requirements. As we mentioned above, recommendation engines and target campaigns can work with the correlated data generated through algorithms. Reuse will help reduce costs and improve RoI for your business.
Virtual Shopping Assistants
While a virtual shopping assistant furthers from the scope of machine learning, it is still built on its fundamentals of it. It is hard to find any business that doesn’t support a chatbot for their platform, but most differ in functionality and possibilities. Chatbots and virtual shopping assistants in retail are one of the most important use cases of machine learning for the industry.
Possibilities of a well-implemented virtual shopping assistant.
Better Navigation
Gone are the days when virtual assistants were only for Q&A. With a good machine learning development partner, you can easily implement navigation, one-touch re-order, a list of popular items in the segment, and more. There are tons of opportunities to move from a chatbot to a full-scale assistant through machine learning.
Decrease in Abandoned Carts
You can start pushing notifications to customers with abandoned carts with the stats generated throughout. The concept ensures that more people complete their purchases irrespective of the time they take.
Cross-Selling
A virtual assistant can be used to cross-sell best sellers generated through a recommendation engine. The technique is more like to induce a sale than just putting recommendations out there.
Enhanced Customer Service
Users across the world are used to immediate response and faster customer services, with the giants already offering so. You can implement better customer service using a chatbot or virtual assistant where queries can answer in a split second.
Improved Data Collection
Users are now more and more comfortable sharing their data with virtual assistants. While a website has a sort/filter option, people would still like recommendations personalized to their liking by a virtual assistant. As an example, imagine a chatbot that can deliver results specific to sorting and filtering, which isn’t available otherwise. It helps users while collecting highly specific data for a variety of improvements for the business.
Customer Profiling
Customer profiling can help your business grow with almost every risk mitigated. You can predict a nearly perfect outcome each time with a good number of users and sales data. Every point we have discussed above uses machine learning in one way or another; for that, you need data. Customer profiling, in essence, is collecting data and using it to improve your business.
Once you have raw data, you can process it through the algorithm to determine the priorities and requirements of a particular customer. You can address their liking and requirements based on their location, time of the year, and whatnot. The possibilities of a successful implementation are simply endless; to know more, you can easily book a free consultation.
Demand Forecasting
Demand forecasting focuses on predicting the future based on current scenarios. While a few methodologies rely on past data and compare it for a better outcome, a more generalized approach can also be implemented. The most common example is if a world cup is coming, more people would be looking forward to buying their favorite team’s jerseys or accessories. However, it is not so simple in the actual scenario, as we need to be much more accurate.
There are four popular ways to forecast demand.
Active Demand Forecasting
It is one of the most popular methods for retailers who do not have data from the past. The method focuses on using outside information, including industry statistics, economic conditions, market conditions, supply chains, and more. Those starting out can leverage this data to produce what’s required for further forecasting.
Delphi Method
The Delphi method is a qualitative analysis using expert opinions on the matter. In case you are planning to enter a market, you can look up or create a questionnaire where experts answer your questions. Market dynamics are highly dependent on this method as people tend to lean towards expert opinions more than other factors.
Barometric Technique
The Barometric technique is a combination of three different factors to give an accurate forecast of the demand. The factors include:
- Leading Indicators – The events that cause the demands to go up or down.
- Coincidental Indicators – The events that happen at the same time as leading events.
- Lagging indicators – The events that happen after the leading indicators have passed.
This is helpful because different unrelated factors are in play, supposedly rain affecting the sales of shoes. The indicators mark and generate correlations between events that are completely different from each other.
Life Cycle Model
It is a model which considers the lifetime of a particular product. There are tons of ways to comprehend its uses, including an invaluable outlook on when the customer would demand a particular product again. The same model can also help reduce deadstock with labels that indicate when a product is supposed to be discarded. The method helps demand forecasting while considering things from the perspective of deadstock and expiration.
Winding Up
We hope you are versed in the popular use cases of machine learning in retail. While we tried to cover the best of it, there may be things left that you would want to discuss. For that, we have decided to offer a free consultation with the experts, and you can avail of it by contacting us. We know the retail industry is far and wide, but with experienced consultants and developers, you can reach unimagined heights.
If you are looking for retail software development, we can ensure it is the most advanced when it comes to analytics. Our developers have immense experience building user-friendly solutions while our ML experts add on the data processing end for groundbreaking results. If you have any questions or would like to discuss possibilities, feel free to contact us, and we will revert to you within 48 business hours.