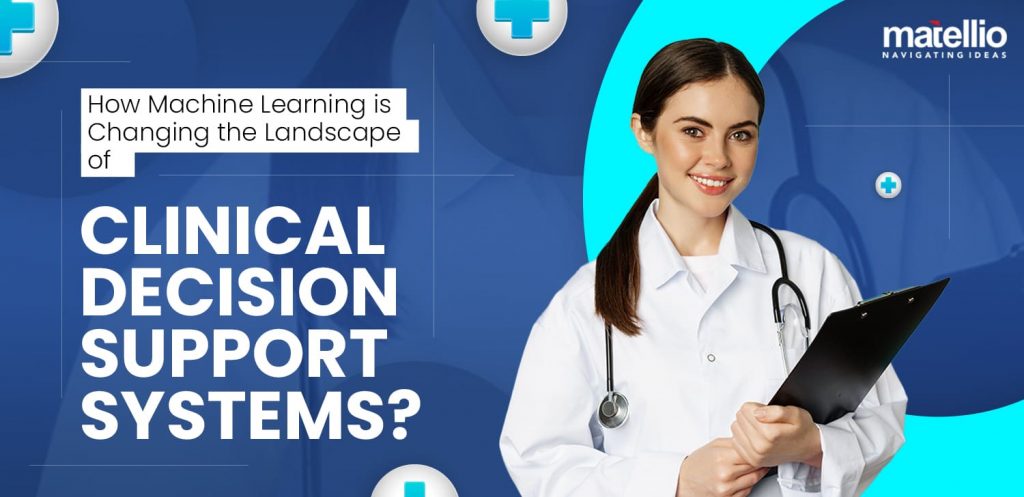
Post-pandemic 2019, healthcare providers across the globe started adopting various digital innovations to offer an improved form of care. It is the reason why clinical decision support systems were quickly adopted within the healthcare industry as an essential tool. This frequent growth can be expressed using the market stats. The global clinical decision support systems market was $1.5 billion in 2022. It is anticipated to be $2.2 billion by 2027 at a CAGR of 7.9%. (SOURCE)
Through this blog, we will be talking about various vital aspects of CDSS and offer you useful insight into the same.
Table of Contents
What are Clinical Decision Support Systems (CDSS)?
CDSS are computer-based systems that assist healthcare professionals in making clinical decisions. They use a combination of patient data, medical knowledge, and clinical guidelines to provide recommendations and alerts to healthcare providers at the point of care. It can improve the accuracy and efficiency of diagnoses, treatment plans, and other clinical decisions and can also help to identify potential health risks and improve patient outcomes. Multiple healthcare providers are hiring healthcare solution development services to bag the best-tailored version of CDSS as per their requirements. It can also be integrated into electronic health records (EHRs) and other clinical systems and can be used in a variety of settings, including hospitals, clinics, and primary care practices.
Advantages of Using Clinical Decision Support System Software
CDSS play an important role in healthcare by assisting healthcare professionals in making clinical decisions. They can help to improve the quality and safety of care by providing accurate, up-to-date, and evidence-based information at the point of care. We have mentioned the market stats for CDSS above showcasing its frequent adoption. What is the reason behind this expansion? Here we have offered you clarity by mentioning a few major perks that are offered by Clinical Decision Support Systems in Healthcare industry.
Enhancing Diagnosis Accuracy
One of the crucial processes that healthcare providers have to handle is diagnosing the patient. With the help of CDSS this process can be completed with improved efficiency. This system helps to identify potential diagnoses and assist in ruling out other potential diagnoses, which can lead to earlier and more accurate diagnoses.
Enhancing Treatment Plans
AI and machine learning in healthcare has added value to the industry and made it more efficient. The CDSS developed using these next-gen technologies has also enhanced its working. It can provide recommendations for treatment options based on the patient’s medical history, test results, and current condition. This ultimately helps to ensure that the patient receives the most appropriate and effective treatment.
Identifying Potential Health Risks
Another advantage that this system has introduced within the healthcare industry has improved the patient safety. CDSS can alert healthcare providers to potential health risks, such as drug interactions or contraindications, which can help to prevent adverse events. Thus, the patient’s safety is increased.
Improving Patient Outcomes
Clinical decision support systems can help to improve patient outcomes by providing real-time support to healthcare providers. It helps in ensuring that patients receive the most appropriate and effective care. The CDSS can be customized as per the requirement and so it can fit various healthcare procedures.
Also Read: How Is Machine Learning Transforming Healthcare?
Cost Savings
With the adoption of CDSS the healthcare providers can offer real-time decisions. It has helped in reducing the number of unnecessary tests that the patients have to go through. The procedures of the required diagnosis can be carried out efficiently. It also minimizes the risk of errors and adverse events, which can lead to cost savings.
Supporting Adherence to Clinical Guidelines
Using the clinical decision support system software can enhance the working of any healthcare provider. It can ensure that the healthcare providers adhere to the most current clinical guidelines and best practices. This can help in improving the quality and consistency of care regularly without any issues.
Applications of Clinical Decision Support System Software
There are numerous processes within the healthcare system in which the CDSS can be integrated. The advantages mentioned above would have given you the idea. To be clear we are providing you with a variety of applications where this system can be used. They are:
Diagnosis and Treatment Planning
Clinical decision support systems can assist healthcare providers in identifying potential diagnoses. It can help rule out other diagnostic processes which are irrelevant to the condition. Not just the diagnosis but it can also provide recommendations for treatment options based on the patient’s medical history, test results, and current condition. It can help to ensure that the patient receives the most appropriate and effective treatment.
Medical Education and Research
CDSS can be used as a teaching tool for medical students, residents and fellows. It can help them understand the reasoning behind a diagnosis or treatment plan. Additionally using this software can also assist the clinical researchers. They can use this system in analyzing large amounts of data, identifying patterns and trends and to generate new hypotheses.
Medication and Chronic Disease Management
Clinical decision support systems in healthcare industry can assist the healthcare providers with the potential drug interactions or contraindications, preventing the adverse events. It can also assist healthcare providers in managing chronic conditions, such as diabetes or heart disease, by providing real-time support at the point of care.
Telemedicine
CDSS can be used in telemedicine settings to provide real-time support and guidance to healthcare providers who are providing care remotely. Even during remote care this system ensures that the healthcare providers adhere to the most current clinical guidelines and best practices, which can help to improve the quality and consistency of care.
Electronic Health Record (EHR) Integration
Clinical decision support system software can be integrated into EHRs and other clinical systems, which can provide healthcare providers with real-time access to patient data and decision-making support at the point of care.
Also Read: A Comprehensive Guide to Electronic Health Record Implementation
Enhance Patient Safety
CDSS have the potential to significantly improve patient safety by providing healthcare providers with timely and accurate information that can help them make better clinical decisions. Some of the ways in which it can enhance patient safety includes:
a) Supporting Clinical Guidelines and Protocols
Using this system can help to support clinical guidelines and protocols. It offers healthcare providers with access to up-to-date, evidence-based information and recommendations. This can help to ensure that patients receive the appropriate care and treatment in accordance with current best practices.
b) Identifying and Managing High-Risk Patients
The clinical decision support systems help the healthcare providers to identify and manage high-risk patients. It can be done easily as this system offers real-time alerts and recommendations for patients who are at risk of developing certain conditions or complications. This can help to ensure that these patients receive the appropriate care and treatment in a timely manner.
c) Reducing Diagnostic Errors
Reduction within the diagnostic errors carried on by the healthcare providers is possible with the help of CDSS. It offers access to the up-to-date, evidence-based information and guidelines. This can help the healthcare providers to make more accurate and timely diagnosis, which can lead to improved patient outcomes.
d) Improving Medication Safety
Another reason why clinical decision support systems and patient safety are kept together is because it improves medication safety. The healthcare providers can bag real-time alerts and recommendations for medication dosing and interactions. This can help to reduce the risk of adverse drug events, such as medication errors and allergic reactions.
e) Enhancing Communication and Coordination
CDSS can help to enhance communication and coordination between healthcare providers by providing them with access to a patient’s complete medical history and by flagging potential issues or concerns. This can help to ensure that all members of the healthcare team are aware of a patient’s condition and can make informed decisions.
Reasons that Make ML Best for Your Clinical Decision Support Systems
If you know even a little bit about the perks offered by machine learning solutions in healthcare you would be aware how useful it can be. Here we have mentioned different ways in which you can analyze how perfect the ML empowered CDSS can be.
Improving the Accuracy of Diagnosis
ML-based CDSS can analyze large amounts of patient data. It can identify patterns and trends that can assist in identifying potential diagnoses and ruling out other potential diagnoses. This can lead to earlier and more accurate diagnoses.
Automating Routine Tasks
Machine learning in the healthcare industry can automate routine tasks such as data entry, within CDSS. This can free up healthcare providers’ time and allow them to focus on more complex and critical tasks.
Enhancing Treatment Recommendations
The CDSS based on machine learning, can analyze patient data and provide recommendations for treatment options. These recommendations are tailored to the patient’s individual needs, which can help to ensure that the patient receives the most appropriate and effective treatment.
Predictive Analytics
The integration of machine learning within CDSS can help the healthcare providers to use the historical patient data for predicting the outcome. For example, the likelihood of a patient developing a certain condition or responding to a certain treatment can be predicted using the past data. This can help healthcare providers to make more informed decisions about care and treatment.
How to Develop Clinical Decision Support Systems with Machine Learning?
After learning about the advantages, applications and other vital concepts, you would be eager to learn about the essential steps used for developing this system. You would be confused about where to start from, or do we need to hire machine learning developer? Worry not, here we have presented some of the basic yet crucial steps that are typically involved in developing CDSS with Machine Learning.
Define the Problem
The first step in developing a CDSS with ML is to clearly define the problem that the system is intended to solve. This may involve identifying the specific clinical decision-making task that the system will support, such as diagnosing a disease or recommending treatment.
Collect and Prepare Data
Once the problem has been defined, the next step is to collect and prepare the data that will be used to train the ML model. This may involve collecting patient data from electronic health records (EHRs) or other sources and then cleaning, preprocessing, and formatting the data so that it is suitable for use in an ML model.
Choose the Team
After the relevant data is gathered it’s time to choose the development team you want to build your CDSS. You can choose to have in-house development, but it can cost you more. Next option is to hire a machine learning development company to create the system as per your need. Try to choose a company that offers experts healthcare technology consulting services so that you get guidance about the suitable trends and technologies for your project development.
Develop the ML Model
The next step is to develop the ML model that will be used to make decisions. This may involve selecting and training an appropriate ML algorithm, such as a decision tree or neural network, on the prepared data. The experts you choose for the development process can help you in choosing the ML model.
Evaluate the Model
Once the ML model has been developed, it is vital to evaluate its performance using a set of test data that was not used during the training phase. This will help to determine how well the model is able to make accurate predictions.
Integrate the Model Into the CDSS
After the ML model has been developed and evaluated, it must be integrated into the clinical decision support system. This may involve building interfaces that allow healthcare providers to access the model and use it to make decisions at the point of care
Test The Integrated System
The next step is to test the integrated system. This may involve testing the system on a small group of real patients to ensure that it is functioning as expected and that the ML model is providing accurate and useful recommendations.
Deploy and Maintain the System
After the system has been tested, it can be deployed and made available to healthcare providers. It is important to continue to monitor and maintain the system to ensure that it is functioning correctly and to make any necessary updates or changes.
During the development procedure, it is important to ensure that the system complies with the regulations and guidelines set by the relevant authorities. Continuous monitoring of the system for ethical, privacy, and security concerns is important. Now you know the major steps involved in developing a CDSS with ML. But you must know that there are two options for the development process: either you can develop a clinical decision support system from scratch by using the advancements of ML. Or you can build the ML model and integrate it within your pre-existing system, depending upon your needs.
Challenges that Require Your Attention!
Despite the many potential benefits of ML-based CDSSs, several challenges must be addressed to realize their full potential.
- Firstly, there is a need for high-quality training data that accurately represents the diverse population of patients.
- Secondly, there is a need for effective methods to validate ML algorithms and ensure their accuracy and reliability.
- Thirdly, there is a need to address the ethical and legal implications of using ML in clinical decision-making, such as ensuring patient privacy and avoiding algorithmic bias.
Lastly, we can say that ML is changing the landscape of clinical decision-support systems by providing healthcare providers with new tools and insights to support more informed and personalized decision-making. However, further research and development are needed to fully realize the potential of ML-based CDSSs and address the challenges that lie ahead.
Conclusion
After scrolling through the entire blog, you would agree that machine learning and clinical decision support systems are evolving. The coming time will offer a well-equipped platform to the healthcare industry and enhance regular care delivery. The ML and AI-fueled tools will raise the healthcare industry’s standards. So, if you wish to upgrade your CDSS by integrating ML or develop a clinical decision support system from scratch for your business, go for it.