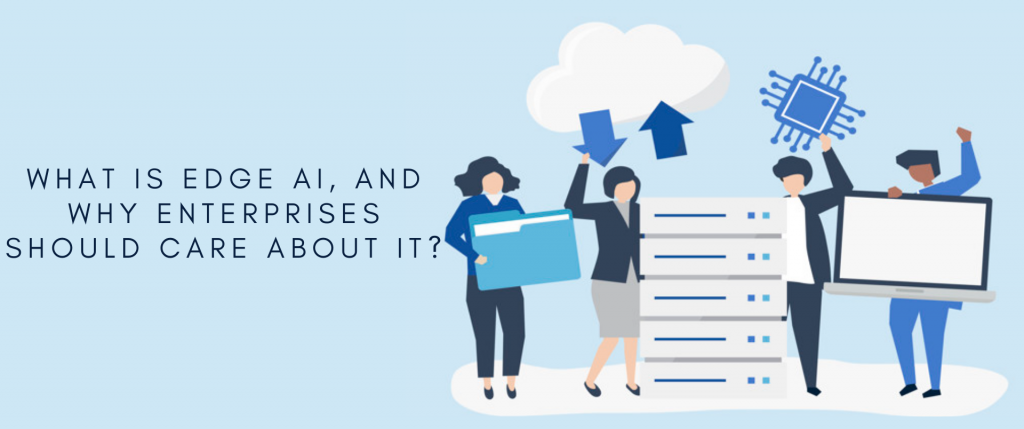
After a Thanksgiving Dinner at my friend’s place, I came back home and said, “Hi Google, turn on the lights”, as usual. And then a reply came promptly, “Sorry, I’m not connected to the internet!”. But wait, what? It wasn’t the lost internet connection that surprised me, but *Why* do I need to be in the dark just because there is lost connectivity? Well, the possible answer is that the AI voice recognition technology runs on the cloud, and lost internet connectivity implies no cloud, and no cloud means no light! But can’t we perform the same locally? As in, without an internet connection? Well, we can, and this is where Edge Technology or to be precise Edge AI steps in. In this article, we will cover the various edges of this advancing technology and how enterprises benefit from this amazing concept.
Edge Computing
Network Edge or simply say Edge is where the data is stored and collected. Excessive power consumption, scalability issues, latency, and connectivity are few of many factors that contribute to the demand for an edge infrastructure.
As per a study by Million Insights, the edge computing marketing is expected to hit $3.24 billion by 2025.
Following Factors contribute to the growth of Edge Computing-
-
Real-Time Customer Engagement
The edge computing technology lets you connect with your customers in real-time, regardless of the location. The fact is very much supported by the varying demand of customers such as Alexa or Siri usage in day-to-day tasks.
-
IoT and Sensor Data Analysis
During the interaction with IoT enabled equipment, all the data from IoT devices like sensors has to be processed and analyzed in milliseconds, without sending them to some off-shore cloud location. From mainframe to cloud, and then to Edge- the shit is massive. But it doesn’t diminish the impact of AI. In fact, it is quite relevant. Technologies like IoT act as an extension of cloud computing only and not the Edge AI has so much to offer as well.
Read More: Don’t Miss Out the Top Edge Computing Use Cases of 2023
What is Edge AI?
Edge AI moves AI closer to where it is required, i.e., in the device itself instead of staying in the cloud. The AI algorithms are processed locally on the device itself, without wanting any connection. It uses the data (generated from the device) and processes it to provide real-time insights.
Does Edge AI really exist?
Yes, it does. There are some popular real-time instances in which the complex algorithms are used to process the data right in your device instead of sending it to cloud for obtaining results-
- iPhone registering and recognizing your face for unlocking the phone in milliseconds.
- Smart refrigerator reminder for purchasing missing dairy stuff.
- And, Google Maps pushing alarms about the bad traffic.
How Edge AI works?
Before we dive deeper into this topic, it is essential to know how ML works. There are two primary phases involved- Training & Inference.
- Training
It is the stage in which a large amount of (known or labeled) data is given to the ML algorithm for it to “Learn.” Using this data, the algo would generate a mode which contains all the results of learning. This phase demands a lot of processing power.
- Inference
It uses the learned model with the new information (processed data) to infer what it should recognize. By going with the example given at the very start of this read- it would be turning off lights.
Known or Labelled data in the training stage is when every data piece has some tag or sticker attached to it, for describing it. AI-powered speech recognition technology is equipped with a lot of voice data for extracting the text from a sentence spoken. NLP techniques then convert this text into commands which a computer can comprehend.
Upon training, the model requires some processing power for performing inference phases.
The primary reason for that is that inference avails a single set of input data, while the training typically expects a large number of samples. The production model (used for inference) is even frozen as it can’t learn anymore and may have less relevant features eliminated for optimizing it for the targeted environment. The final result is that it can directly work on an embedded device, i.e., The Edge. This process passes the decision power to the device and allows it to be autonomous. This is what is called Edge Artificial Intelligence.
What does Market Statistics have to say in this context?
The market for AI is on the rise. As per research, a total of 3.2 billion devices will be shipped with AI-enabled capabilities by the year 2023.
A report by Tractica states that the shipping of AI edge devices is expected to rise from 161.4 million units (in 2018) to 2.6 billion units (by 2025). As per the analysis, the top AI-enable edge devices in terms of volume are as follows-
- Smart Speakers
- Mobile Phones
- Drones
- Consumer and Enterprise Robots
- Automotive
- Security Cameras
- Head-Mounted Displays
- PCs/Tablets
Comparison between Cloud AI and Edge AI
Now let’s see how differently Edge AI and Cloud AI are positioned in the market- the propositions they offer, and the business models they retain.
Cloud AI
Cloud AI is provided as a service by either charging for the amount of data processed and trained on a pay-per-use or subscription model or value-added services such as infrastructure planning, cybersecurity, process monitoring, and much more.
Amazon, IBM, Google, Oracle, Facebook, Alibaba are some of the established players in the cloud AI market. Certain small players offer specialized cloud AI services for addressing specific verticals and use cases.
Advantages of Cloud AI
- Can process large volumes of variable data.
- Offers high velocity of data processing.
- Supercomputing capabilities.
- Offers reliable and efficient training.
Disadvantages of Cloud AI
- Dependent on the connectivity between cloud and devices.
- Possess a higher cost
- Protection and Data privacy can be an issue.
Edge AI
We have already discussed Edge AI in detail above, but now we are going to see the three implementation models of Edge AI-
-
On-Premise AI server
The implementation is dependent upon the software licensing and is usually designed for supporting several use cases.
-
Intelligent Gateways
In this one, the AI is executed at a localized gateway to the edge device.
-
On Device
This implementation mode refers to the hardware which can execute AI training and inference functions of the device. The training phase could be devolved here, while the inference is made on the device itself.
Advantages of Edge AI
- Inference is done in almost real-time, on edge.
- No connectivity issues as inference are always on.
- Speed of processing.
- Retention of data at the device.
- Low Latency for mission-critical tasks.
- Has lower AI implementation costs.
Disadvantages of Edge AI
- Requires in-house skills and knowledge.
- Requires resources for Data Processing.
Why should Enterprises care about Edge AI?
Edge AI solutions offer no time-lapse or latency between the analysis and storage of data. The decisions are delivered right from the derived insights- empowering analytics and IoT.
Costs are no longer a challenging attribute, as the Edge requires lesser connectivity for transferring data.
Data is the new platinum or gold, but it has its peculiarities. But mostly, enterprises are incapable of deriving the best out of the reams of data. It is because the real value of data lies in the combination of data sets from multiple devices for deriving patterns that can aid in predicting future trends. The complex algos can use this data as input and process it using powerful computing devices like GPUs. Enterprises can create patterns that are way more insightful than basic analytics.
Edge AI provides more accurate data pertaining to varied business scenarios that support better decision making. GPUs are also being deployed increasingly for raising the abilities of AI.
For enterprises, AI-enabled IoT devices that collate data from both the software and hardware stack help the operational technology in delivering real-time, intelligent decisions for the business. With the hive-like structure, Edge AI has the ability to shift collection, analysis, and storage of data, for keeping it away from the cloud to have it all light & agile.
Another major advantage enterprises entertain its quickly implementing mitigation tactics. The process involves a thorough risk analysis for identifying possible entry points for attackers.
AI-enabled solutions can proactively detect and prevent cyber-attacks even before they gain access to the cloud.
However, no coin is one-sided. In comparison to a cloud infrastructure, Edge can suffer from the limited computing powers- the fact giving all the advantages as mentioned above a back seat. Although the gap can be filled with new development. Chip manufacturers can cover this up by availing purpose-built accelerators for accelerating the inference process.NVIDIA Jetson, Myriad Chips, Intel Movidius are some of the known brands that provide highly optimized edge pipeline workflow. Enterprise can use this support increase in the upcoming years.
The combination of software platforms and hardware accelerators ensures the efficient running of the inference models on the Edge.
Additionally, the IoT applications for enterprises often come with a hefty workload. The cloud may not be able to sustain it for several industries (manufacturing, medical devices, transportation, etc.) because of this heavy data. The Edge devices equipped with better computing power and storage can support reliable operational infrastructure.
Overall, it has made operations smarter and efficient.
The Verdict
The Edge AI Summit took place in December 2019 at San Francisco, USA. The event had discussed various opportunities and challenges of distributed intelligence and explored the newly found AI adoption round the globe. In an interview with fellow VP and CTO Watson, IBM said that the traditional devices are empowered. Innovative technologies, like 5G networks, offer new opportunities. Due to all this, consumers are expecting better experience and data protection. This, in turn, helps in deriving the need to move analytics and AI closer to where data is generated, and the user experience is delivered.
By stepping into the mainstream adoption, Edge AI shall grow at a CAGR of 41%. Companies will continue to adopt edge strategies for improving their operations and enabling real-time performance by making their systems smart.
The concept of Edge AI is much broader now, and the bandwidth shall keep increasing in the upcoming years.
Hence, it’s time to embrace this new technology with open arms as the future is undoubtedly much more speedier, smarter, and on the Edge!